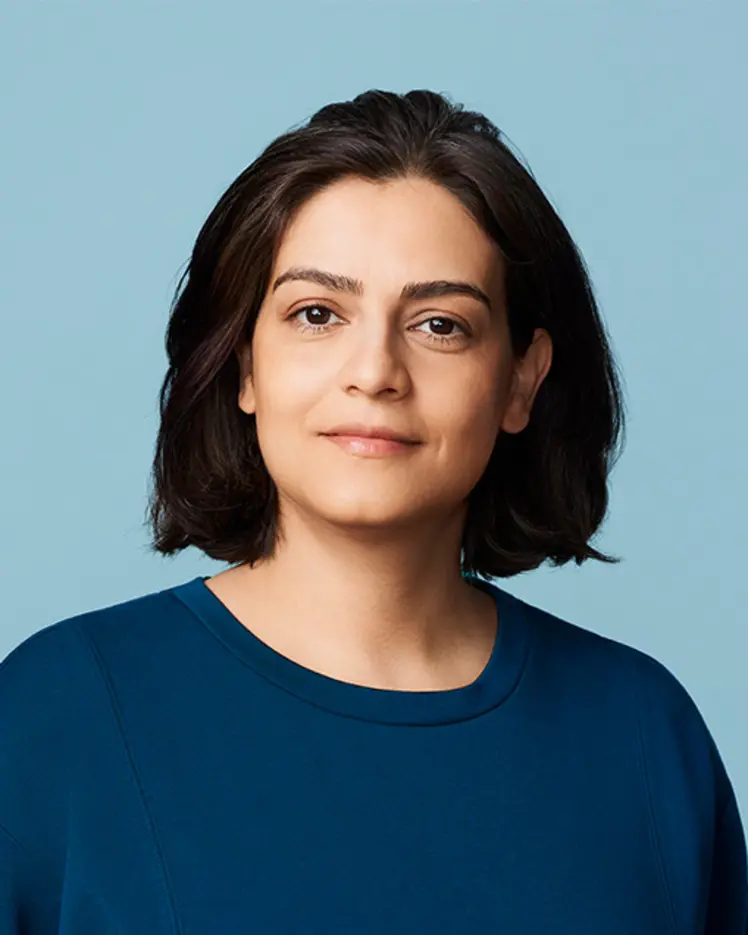
Reihaneh Rabbany
Biographie
Reihaneh Rabbany est professeure adjointe à l'École d'informatique de l'Université McGill. Elle est membre du corps professoral de Mila – Institut québécois d’intelligence artificielle et titulaire d'une chaire en IA Canada-CIFAR. Elle est également membre du corps enseignant du Centre pour l’étude de la citoyenneté démocratique de McGill. Avant de se joindre à l’Université McGill, elle a été boursière postdoctorale à la School of Computer Science de l'Université Carnegie Mellon. Elle a obtenu un doctorat à l’Université de l’Alberta, au Département d'informatique. Elle dirige le laboratoire de données complexes, dont les recherches se situent à l'intersection de la science des réseaux, de l'exploration des données et de l'apprentissage automatique, et se concentrent sur l'analyse des données interconnectées du monde réel et sur les applications sociales.