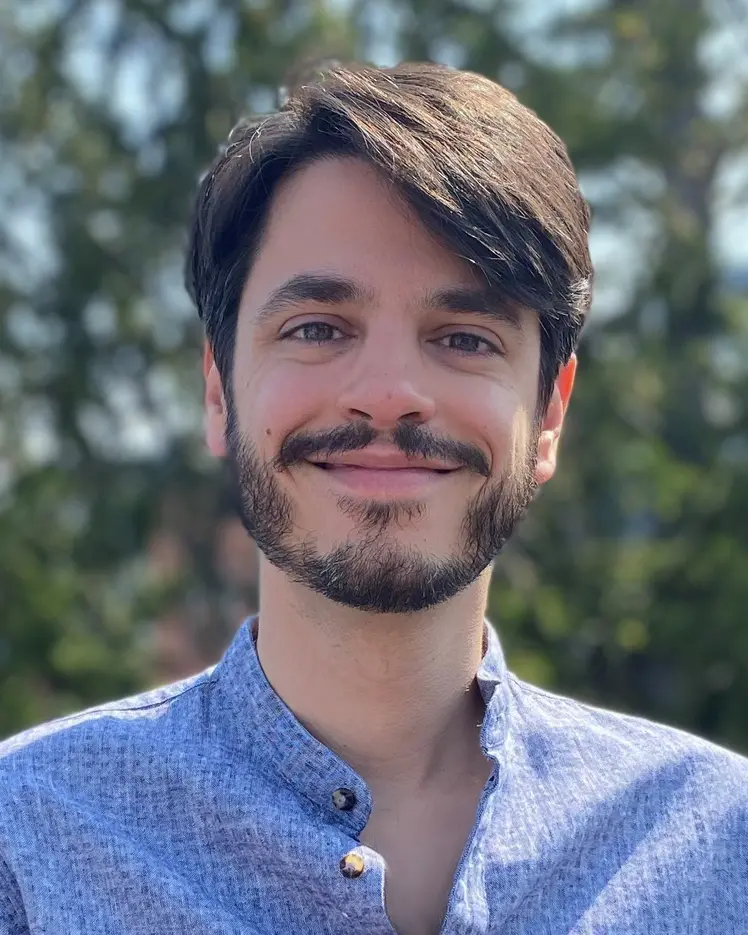
Marco Bonizzato
Biography
Marco Bonizzato is a control, electrical and life sciences engineer with over ten years’ experience in implantable brain-computer interfaces and neuromodulation technology. He has unique double expertise in (a) neural prostheses, and (b) machine intelligence and optimization.
Bonizzato is an assistant professor of electrical engineering at Polytechnique Montréal and an adjunct professor of neuroscience at Université de Montréal.
He also directs the Polytechnique’s sciNeurotech Lab, whose research goal is to develop the entire translational arc of new neurostimulation therapies aimed at restoring sensorimotor function after neurotrauma—from discovery in rodents to application in human medical technologies tailored and personalized to the user using AI.