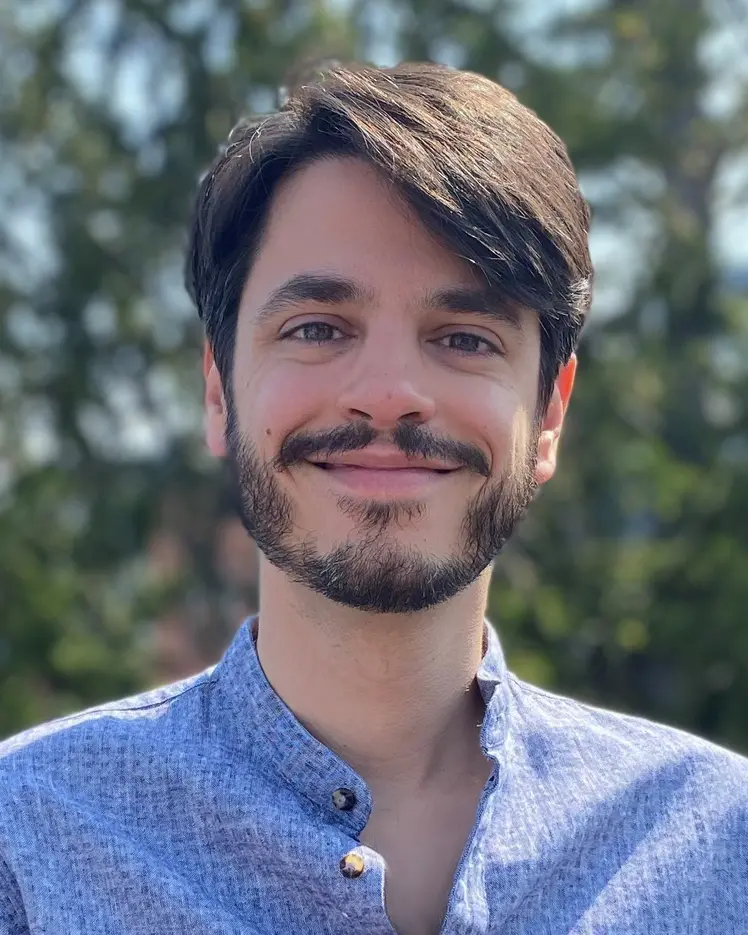
Marco Bonizzato
Biographie
Marco Bonizzato est ingénieur en contrôle, en électricité et en sciences de la vie, et cumule plus de 10 ans d'expérience dans le domaine des interfaces cerveau-ordinateur implantables et de la technologie de neuromodulation. Il possède une double expertise unique en prothèses neurales et en intelligence et optimisation des machines.
Il est professeur adjoint de génie électrique à Polytechnique Montréal et professeur auxiliaire de neurosciences à l'Université de Montréal.
Il dirige également le laboratoire sciNeurotech. L'objectif de la recherche qui y est menée est de développer l'arc translationnel complet de nouvelles thérapies de neurostimulation afin de restaurer la fonction sensorimotrice après un neurotraumatisme, depuis la découverte chez le rongeur jusqu'à l'application dans la technologie médicale humaine, adaptée et personnalisée à chaque utilisateur par l'intelligence artificielle.