Language and Image
Artificial intelligence (AI) systems can process data collected from multiple sources, through a variety of sensors, to help computers make predictions and decisions. Mila’s researchers are pioneers in the fields of natural language processing and computer vision, and continue to explore the intersections of both technologies.
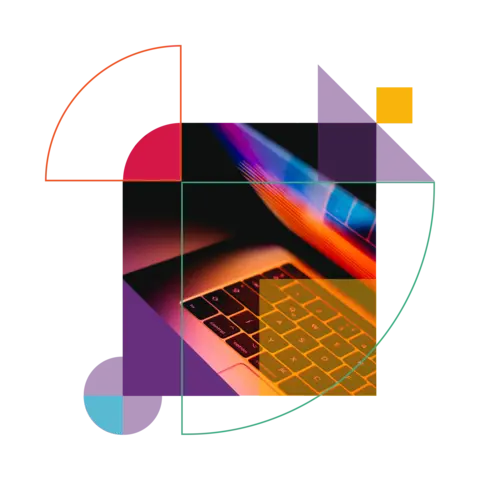