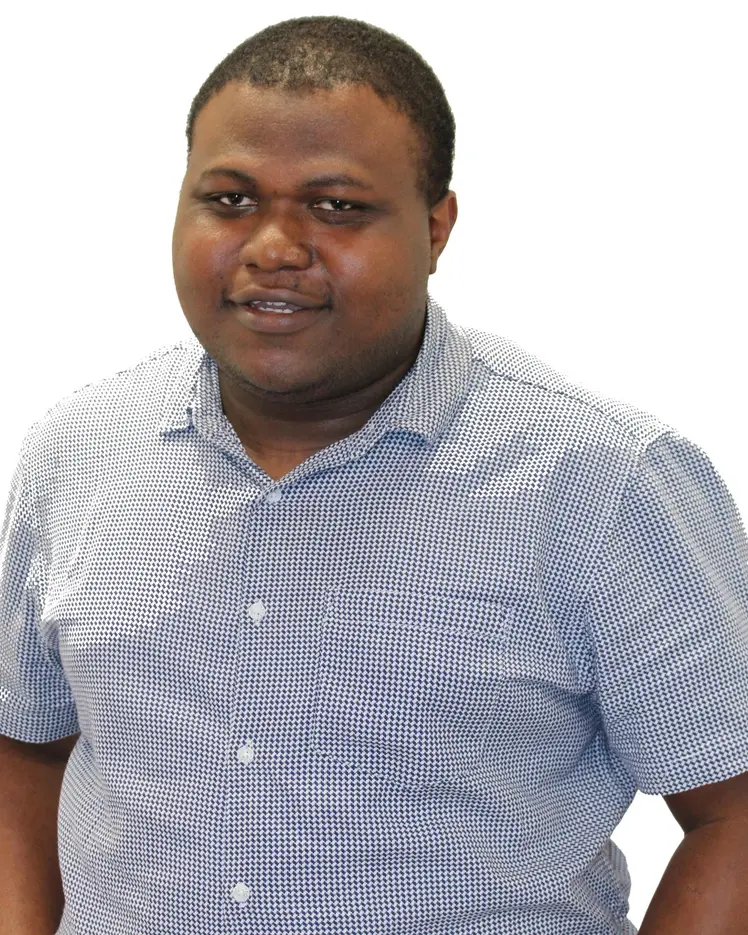
David Ifeoluwa Adelani
Core Academic Member
Canada CIFAR AI Chair
McGill University
Research Topics
Deep Learning
Natural Language Processing
Representation Learning
Biography
David Adelani is an assistant professor at McGill University’s School of Computer Science under the Fighting Inequities initiative, and a core academic member of Mila – Quebec Artificial Intelligence Institute.
Adelani’s research focuses on multilingual natural language processing with special attention to under-resourced languages.