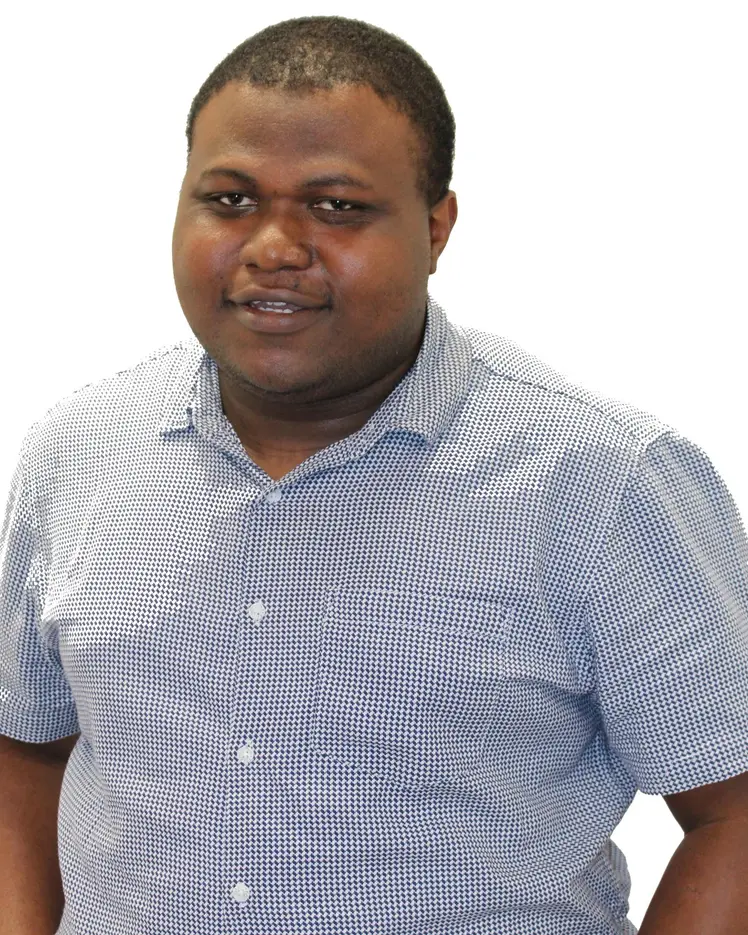
David Ifeoluwa Adelani
Biographie
David Adelani est professeur adjoint en science informatique et lutte contre les inégalités à l’Université McGill, et membre académique principal à Mila – Institut québécois d'intelligence artificielle. Ses recherches se concentrent sur le traitement multilingue du langage naturel, avec un accent particulier sur les langues sous-dotées en ressources.