Environment and Energy
Around the world, people are being increasingly affected by climate change. Through thoughtful interdisciplinary partnerships, artificial intelligence (AI) can become a powerful tool in reducing greenhouse gas emissions, while also helping society adapt to the many climate-based challenges it will face in the decades ahead.
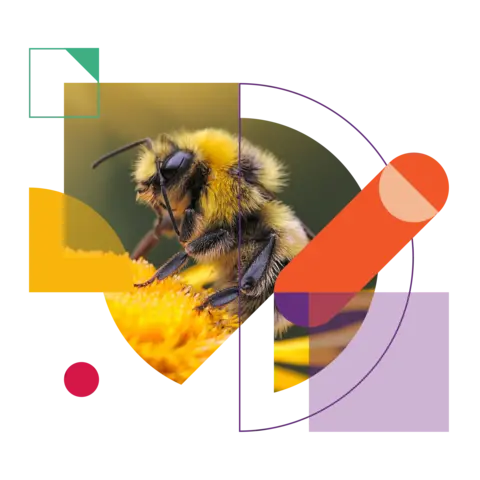