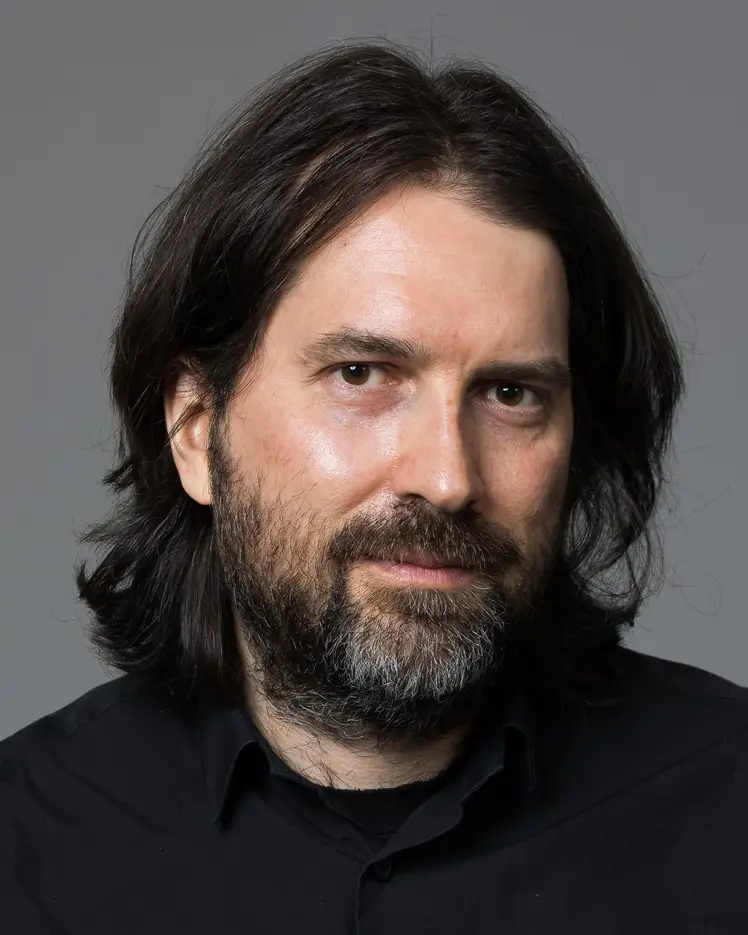
Christian Gagné
Biography
Christian Gagné has been a professor in the Department of Electrical and Computer Engineering at Université Laval since 2008.
He is the director of the Institute Intelligence and Data (IID), holds a Canada CIFAR AI Chair, and is an associate member of Mila – Quebec Artificial Intelligence Institute.
Gagné is also a member of Université Laval’s Computer Vision and Systems Laboratory (LVSN), as well as its Robotics, Vision and Machine Intelligence Research Centre (CeRVIM) and its Big Data Research Centre (CRDM). He is a member of the REPARTI and UNIQUE strategic clusters of the FRQNT, the VITAM centre of the FRQS, and the International Observatory on the Societal Impacts of AI and Digital Technologies (OBVIA).
Gagné’s research focuses on the development of methods for machine learning and stochastic optimization. In particular, he is interested in deep neural networks, representation learning and transfer, meta-learning and multitasking. He is also interested in optimization approaches based on probabilistic models and evolutionary algorithms, including black-box optimization and automatic programming. An important part of his work is the practical application of these techniques in fields like computer vision, microscopy, healthcare, energy and transportation.