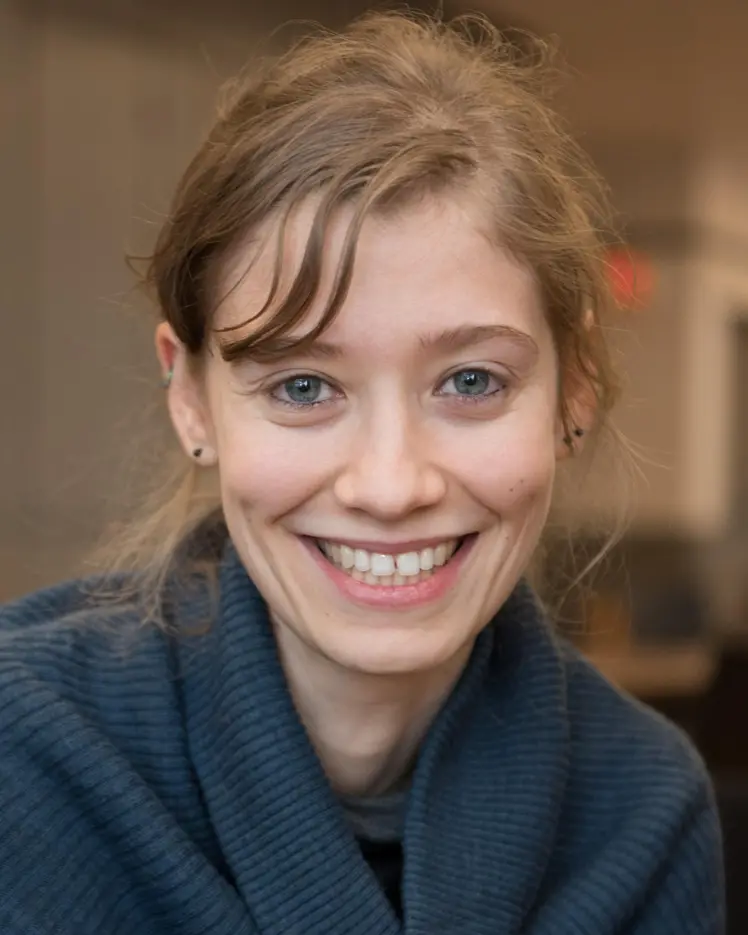
Margarida Carvalho
Biography
Margarida Carvalho holds a bachelor’s and master’s degree in mathematics. She completed her PhD in computer science at the University of Porto, for which she received the 2018 EURO Doctoral Dissertation Award.
In 2018, Carvalho was appointed assistant professor in the Department of Computer Science and Operations Research at Université de Montréal, where she holds the FRQ-IVADO Research Chair in Data Science for Combinatorial Game Theory.
She is an expert in operations research, in particular, combinatorial optimization and algorithmic game theory. Her research is motivated by real-world decision-making problems that involve the interaction of multiple agents, such as kidney exchange programs, school choice and competitive markets.