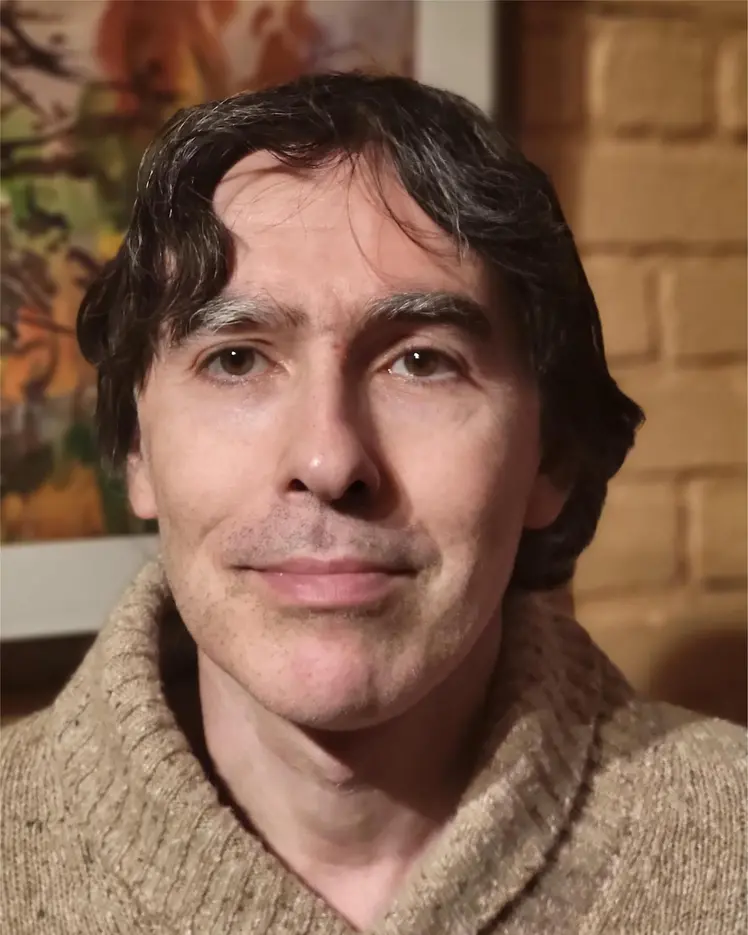
Vladimir Makarenkov
Biography
Vladimir Makarenkov is a full professor and director of the graduate program in bioinformatics at Université du Québec à Montréal (UQAM). He holds a master's degree in applied mathematics from Lomonosov Moscow State University and a PhD in computer science and mathematics from the École des hautes études en sciences sociales (EHESS) in Paris. Before joining the computer science department at UQAM, he completed a three-year postdoctoral fellowship at the Digital Ecology Lab at Université de Montréal.
He is the author of 80 journal articles and 67 conference papers, and the recipient of the prestigious Simon Régnier Prize and the Chikio Hayashi Prize awarded by the International Society for Mathematical Classification. His research focuses on AI, bioinformatics and data mining. This encompasses the design and development of novel unsupervised and supervised machine learning methods, as well as the use of machine learning techniques, including clustering and deep learning, for the analysis of biological and biomedical data.
Makarenkov’s current research also involves the development of an automated recommendation system based on deep learning to recommend the best clustering algorithm for a given input dataset. Additionally, he is working on creating a generic machine learning model to define the concept of cluster, and on comparing various auto-encoding approaches and clustering algorithms to achieve better clustering results.