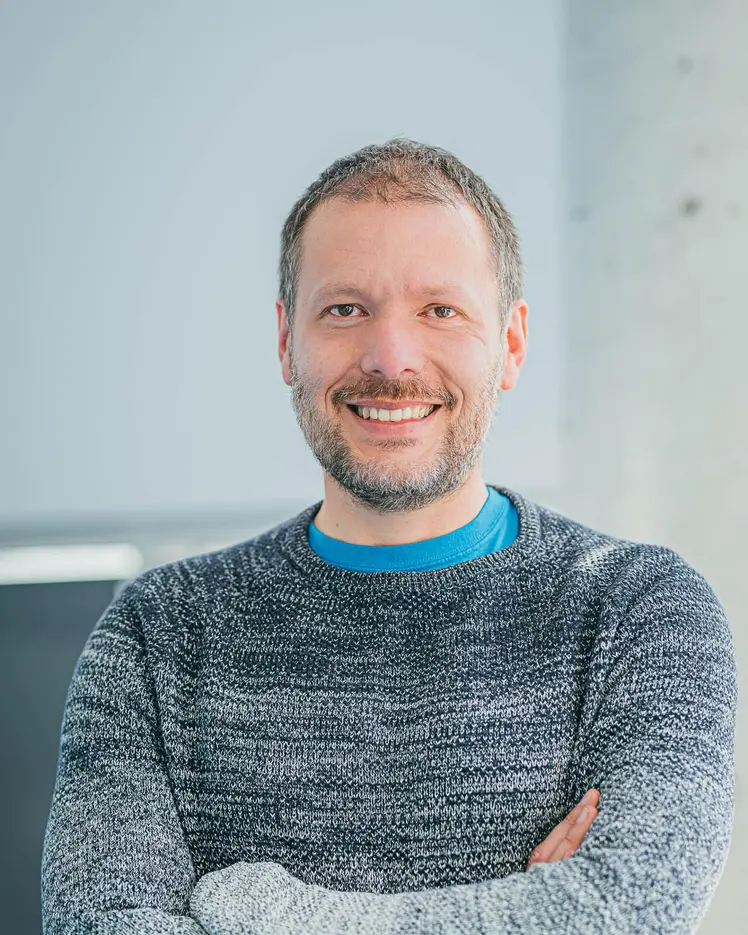
Giovanni Beltrame
Biography
Giovanni Beltrame obtained his PhD in computer engineering from Politecnico di Milano in 2006, after which he worked as a microelectronics engineer at the European Space Agency on a number of projects, from radiation-tolerant systems to computer-aided design.
In 2010, he moved to Montréal, where he is currently a professor at Polytechnique Montréal in the Computer and Software Engineering Department.
Beltrame directs the Making Innovative Space Technology (MIST) Lab, where he has more than twenty-five students and postdocs under his supervision. He has completed several projects in collaboration with industry and government agencies in the area of robotics, disaster response and space exploration. He and his team have participated in several field missions with ESA, the Canadian Space Agency (CSA) and NASA, including BRAILLE, PANAGAEA-X and IGLUNA.
His research interests include the modelling and design of embedded systems, AI and robotics, and he has published his findings in top journals and conferences.