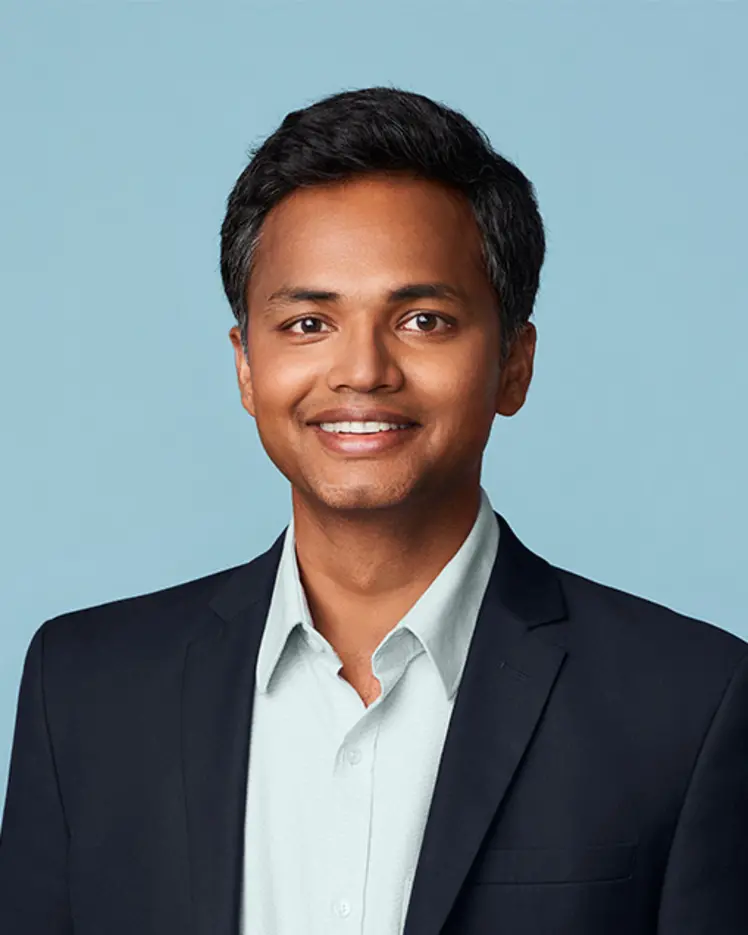
Siva Reddy
Biography
Siva Reddy is an assistant professor at the School of Computer Science and in the Department of Linguistics at McGill University. He completed a postdoc with the Stanford NLP Group in September 2019.
Reddy’s research goal is to enable machines with natural language understanding abilities in order to facilitate applications like question answering and conversational systems. His expertise includes building symbolic (linguistic and induced) and deep learning models for language.