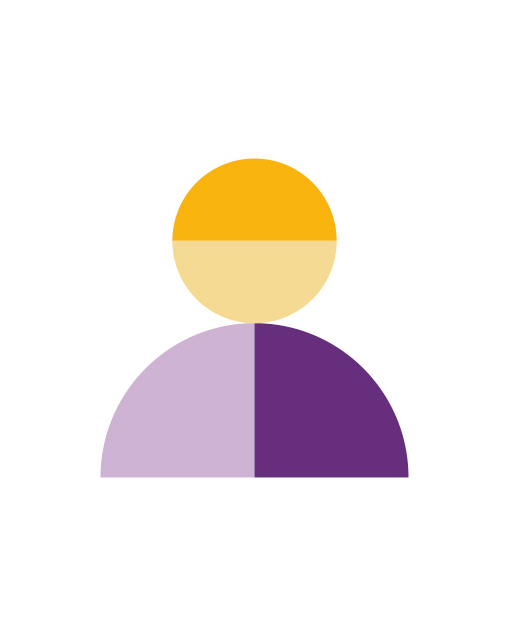
Adriana Romero Soriano
Biographie
Adriana Romero-Soriano est chercheuse à Meta (FAIR, Fundamental AI Research), professeure adjointe à l'Université McGill, membre industriel principal de Mila – Institut québécois d’intelligence artificielle et titulaire d'une chaire en IA Canada-CIFAR. Ses recherches se situent à l'intersection des modèles génératifs, de la vision par ordinateur et de l'IA responsable. Ses travaux les plus récents portent sur l'amélioration de la qualité, de la contrôlabilité, de la cohérence et de la diversité de représentation des systèmes de création de contenu visuel. Elle a obtenu son doctorat à l'Université de Barcelone, où elle a travaillé avec Carlo Gatta, et a été chercheuse postdoctorale pendant deux ans à Mila, où elle a travaillé avec le professeur Yoshua Bengio.