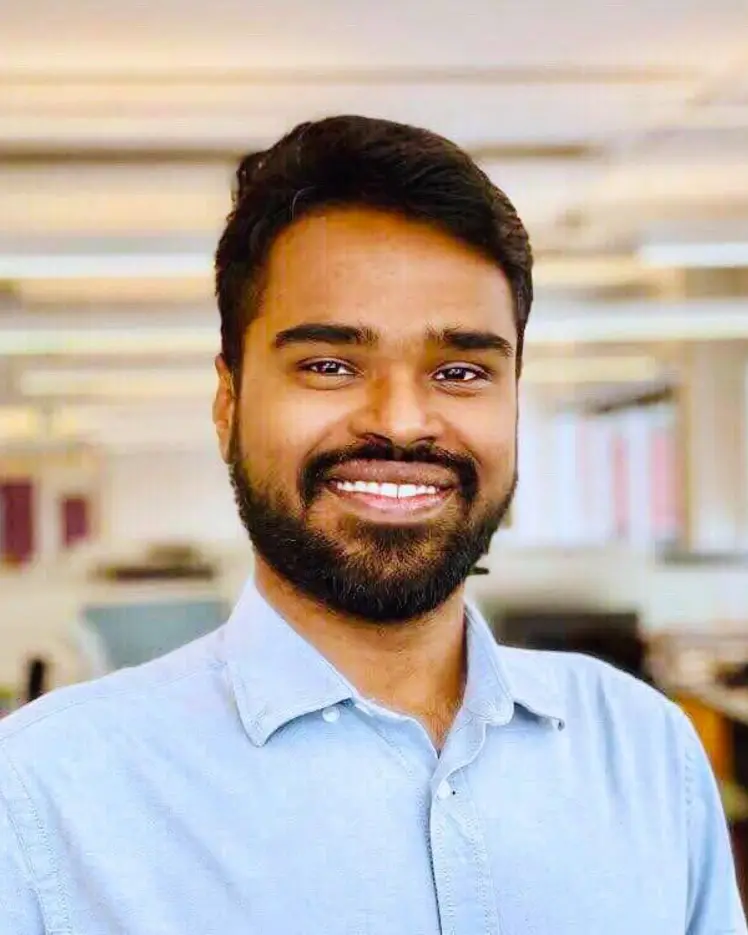
Sarath Chandar
Biographie
Sarath Chandar est professeur associé au départment de génie informatique et génie logiciel de Polytechnique Montréal, où il dirige le laboratoire de recherche Chandar. Il est également membre académique principal à Mila – Institut québécois d’intelligence artificielle, et titulaire d'une chaire en IA Canada-CIFAR et d'une Chaire de recherche du Canada en apprentissage machine permanent.
Ses recherches portent sur l'apprentissage tout au long de la vie, l'apprentissage profond, l'optimisation, l'apprentissage par renforcement et le traitement du langage naturel. Pour promouvoir la recherche sur l'apprentissage tout au long de la vie, Sarath Chandar a créé la Conférence sur les agents d'apprentissage tout au long de la vie (CoLLAs) en 2022 et a présidé le programme en 2022 et en 2023. Il est titulaire d'un doctorat de l'Université de Montréal et d'une maîtrise en recherche de l'Indian Institute of Technology Madras.