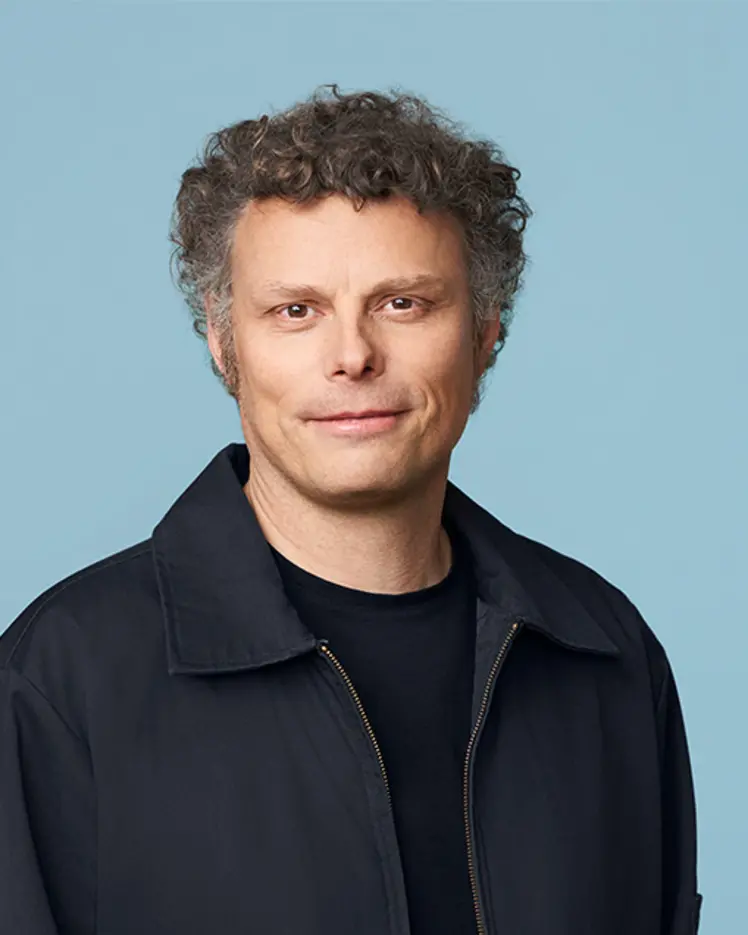
Chris Pal
Biographie
Christopher Pal est titulaire d'une chaire en IA Canada-CIFAR, professeur titulaire à Polytechnique Montréal et professeur adjoint au Département d'informatique et de recherche opérationnelle (DIRO) de l'Université de Montréal. Il est également chercheur émérite à ServiceNow Research. Il est engagé dans la recherche sur l'intelligence artificielle et l'apprentissage automatique depuis plus de 25 ans, publiant souvent des travaux sur les méthodes de modélisation du langage à grande échelle et les techniques de modélisation générative. Il a obtenu un doctorat en informatique à l'Université de Waterloo.