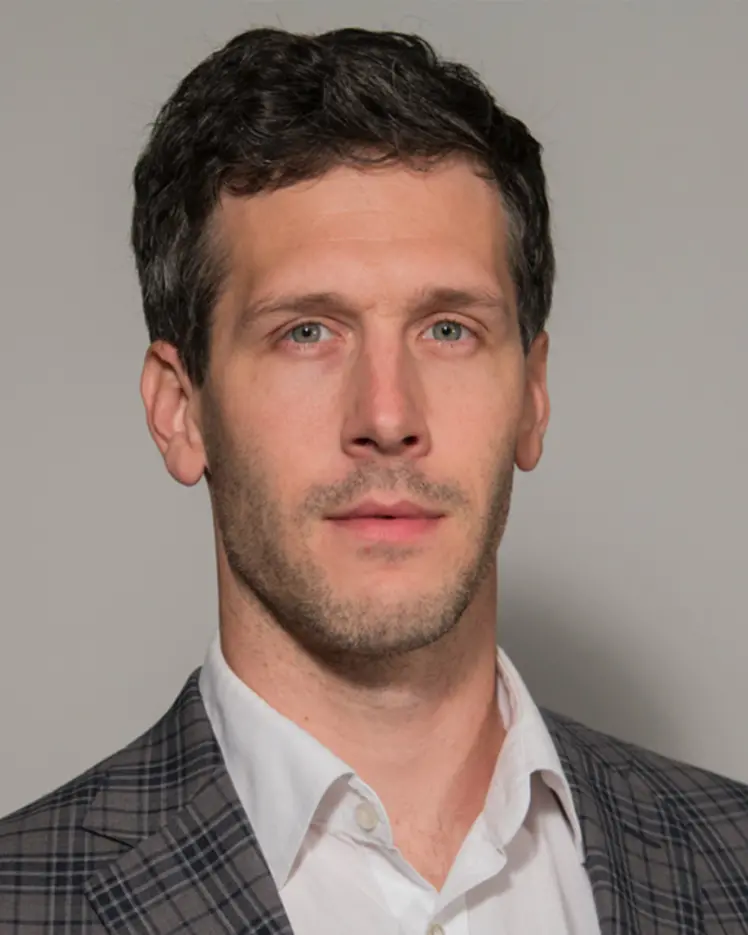
Liam Paull
Biographie
Liam Paull est professeur adjoint à l'Université de Montréal et codirige le Laboratoire de robotique et d’IA intégrative de Montréal (REAL). Son laboratoire se concentre sur les problèmes de robotique, y compris la construction de représentations du monde (pour la localisation et la cartographie simultanées, par exemple), la modélisation de l'incertitude et la construction de meilleurs flux de travail pour enseigner de nouvelles tâches aux agents robotiques (notamment par la simulation ou la démonstration). Auparavant, Liam Paull a été chercheur au Computer Science and Artificial Intelligence Laboratory (CSAIL) du Massachusetts Institute of Technology (MIT), où il a dirigé le projet de voiture autonome financé par le Toyota Research Institute (TRI). Il a également été chercheur postdoctoral au laboratoire de robotique marine du MIT, où il a travaillé sur la technique SLAM (Simultaneous Localization and Mapping) pour les robots sous-marins. Il a obtenu son doctorat en 2013 à l'Université du Nouveau-Brunswick : il s’y est intéressé à la planification robuste et adaptative pour les véhicules sous-marins. Il est cofondateur et directeur de la Fondation Duckietown, dont l'objectif est de rendre accessibles à tous·tes les expériences d'apprentissage de la robotique.