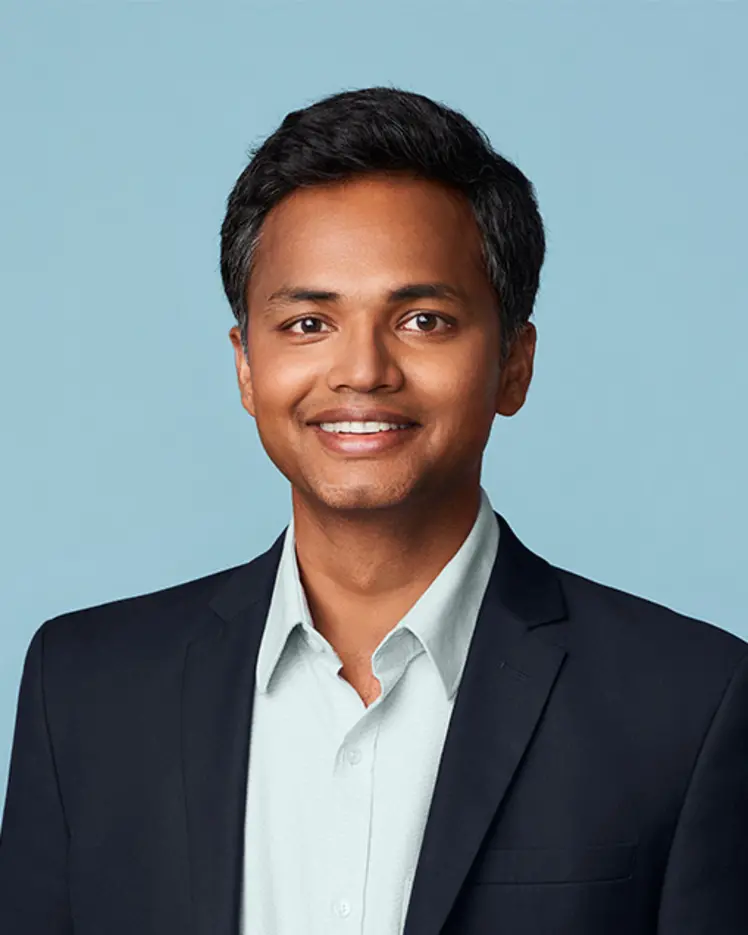
Siva Reddy
Biographie
Siva Reddy est professeur adjoint en informatique et linguistique à l’Université McGill. Ses travaux portent sur les algorithmes qui permettent aux ordinateurs de comprendre et de traiter les langues humaines. Il a fait ses études postdoctorales avec le Stanford NLP Group. Son expertise inclut la construction de symboliques linguistiques et induites et de modèles d’apprentissage profond pour le langage.