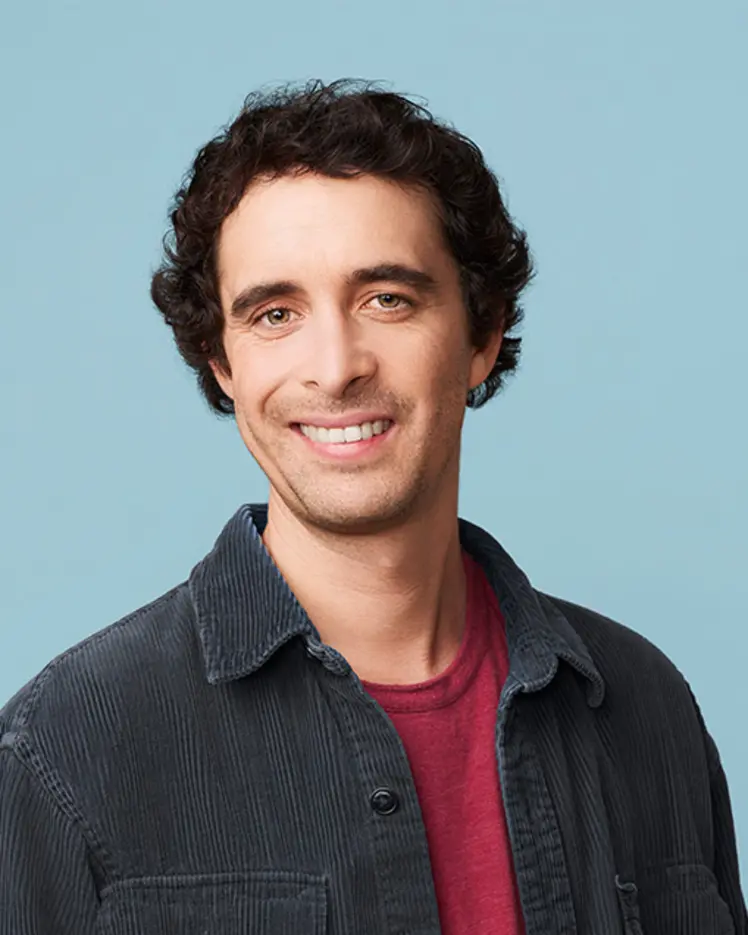
Pierre-Luc Bacon
Biographie
Pierre-Luc Bacon est professeur agrégé au Département d'informatique et de recherche opérationnelle de l'Université de Montréal. Il est également membre de Mila – Institut québécois d’intelligence artificielle et d’IVADO et titulaire d'une chaire Facebook-CIFAR. Il dirige un groupe de recherche qui travaille sur le défi posé par la malédiction de l'horizon dans l'apprentissage par renforcement et le contrôle optimal.