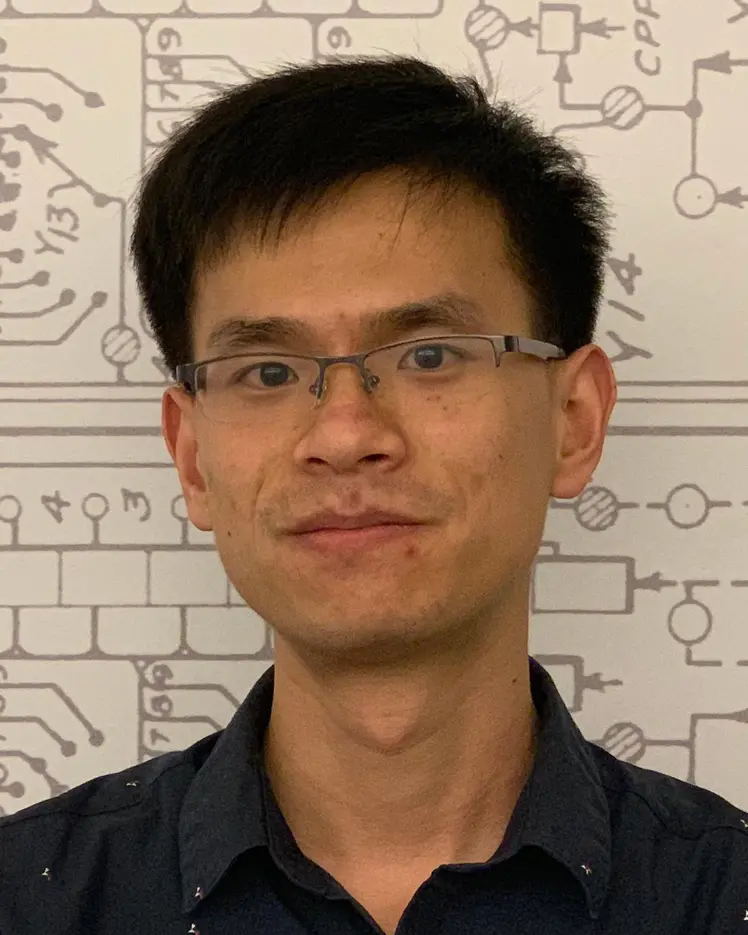
Xujie Si
Biography
Xujie Si is an assistant professor in the Department of Computer Science, University of Toronto. He is also an affiliate member at Vector Institute and an affiliate member at Mila – Quebec Artificial Intelligence Institute, where he holds a Canada CIFAR AI Chair.
Si received his PhD from the University of Pennsylvania in 2020, his master's degree from Vanderbilt University, and his bachelor's degree (with Honors) from Nankai University.
Si’s research lies at the intersection of programming languages and AI. He is generally interested in developing learning-based techniques to help programmers build better software with less effort, integrating logic programming with differentiable learning systems for interpretable and scalable reasoning, and leveraging programming abstractions for reliable and data-efficient learning.
His work has been honoured with an ACM Special Interest Group on Programming Languages (SIGPLAN) Distinguished Paper Award, as well as highlighted at top conferences on programming languages and machine learning.