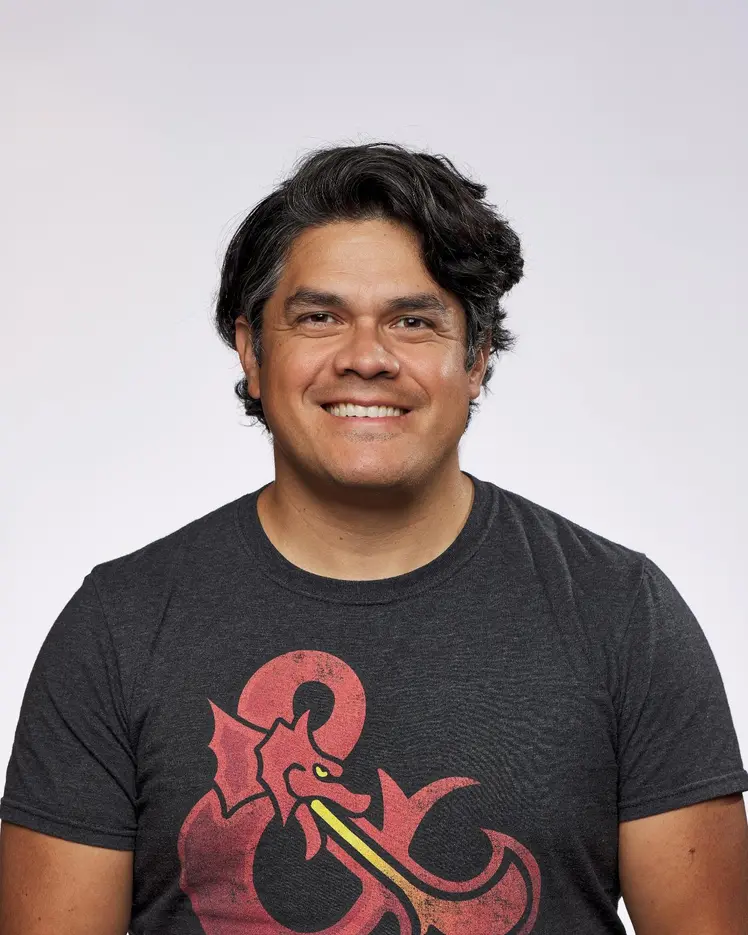
Pablo Samuel Castro
Biographie
Pablo Samuel Castro est né et a grandi à Quito, en Équateur, et a déménagé à Montréal après l'école secondaire pour étudier à l’Université McGill. Il y a obtenu un doctorat en se concentrant sur l'apprentissage par renforcement, sous la supervision de Doina Precup et Prakash Panangaden. Il est chercheur scientifique à Google DeepMind à Montréal. Il s’intéresse particulièrement à la recherche fondamentale sur l'apprentissage par renforcement et plaide régulièrement en faveur d'une augmentation de la représentation des personnes d’origine latino-américaine dans la communauté de recherche. Il est également professeur adjoint au Département d'informatique et de recherche opérationnelle (DIRO) de l'Université de Montréal. Outre son intérêt pour le codage, l'intelligence artificielle et les mathématiques, Pablo Samuel est un musicien actif.