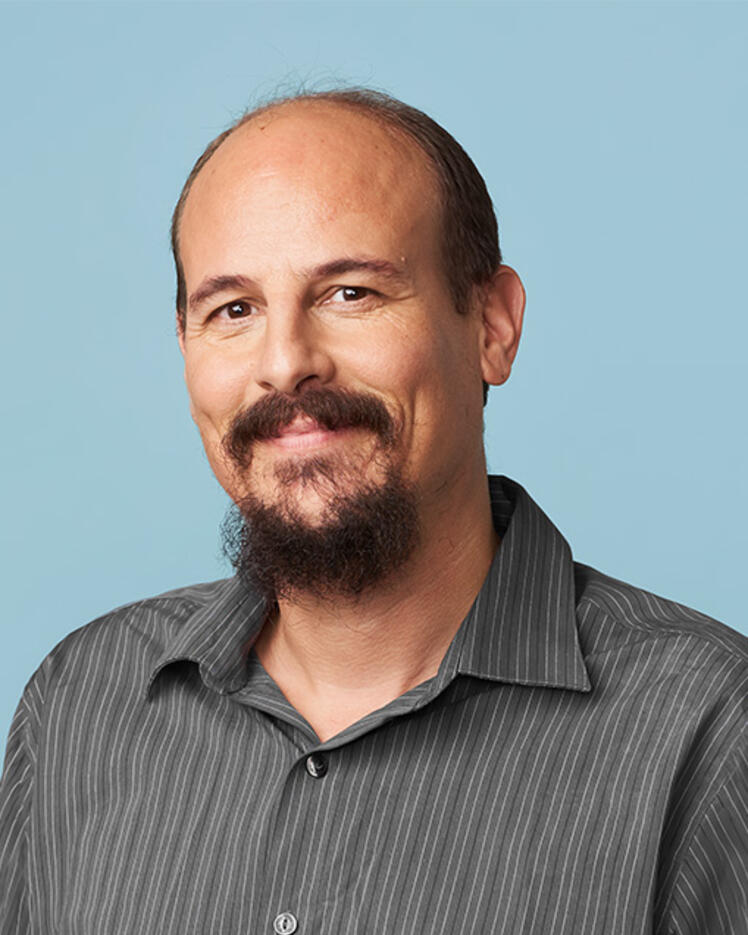
Guillaume Rabusseau
Biographie
Depuis septembre 2018, je suis professeur adjoint à Mila – Institut québécois d’intelligence artificielle et au Département d'informatique et de recherche opérationnelle (DIRO) de l'Université de Montréal (UdeM). Je suis titulaire d’une chaire de recherche en IA Canada-CIFAR depuis mars 2019. Avant de me joindre à l’UdeM, j’ai été chercheur postdoctoral au laboratoire de raisonnement et d'apprentissage de l'Université McGill, où j'ai travaillé avec Prakash Panangaden, Joelle Pineau et Doina Precup.
J'ai obtenu mon doctorat en 2016 à l’Université d’Aix-Marseille (AMU), où j'ai travaillé dans l'équipe Qarma (apprentissage automatique et multimédia), sous la supervision de François Denis et Hachem Kadri. Auparavant, j'ai obtenu une maîtrise en informatique fondamentale de l'AMU et une licence en informatique de la même université en formation à distance.
Je m'intéresse aux méthodes de tenseurs pour l'apprentissage automatique et à la conception d'algorithmes d'apprentissage pour les données structurées par l’utilisation de l'algèbre linéaire et multilinéaire (par exemple, les méthodes spectrales).