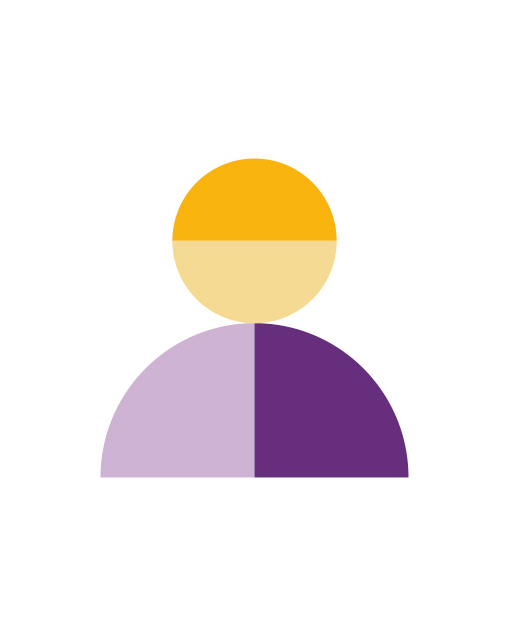
Adam M. Oberman
Biographie
Je suis professeur à l'Université McGill, au sein du Département de mathématiques et de statistiques. Mes recherches portent sur l'application de techniques mathématiques avancées dans le domaine de l'apprentissage profond. Mes principaux champs d'expertise incluent la modélisation générative, les méthodes d'optimisation stochastiques, l'équité et la suppression des biais en matière de vision par ordinateur, et la généralisation dans l'apprentissage par renforcement.
Avant de me joindre à McGill, en 2012, j'ai occupé un poste de professeur titulaire à l'Université Simon Fraser et j'ai effectué un stage postdoctoral à l'Université du Texas à Austin. J'ai réalisé ma formation de premier cycle à l'Université de Toronto et j'ai poursuivi des études supérieures à l'Université de Chicago. Au cours de ma carrière, j'ai également occupé des postes de visiteur à l’Université de Chicago à Los Angeles (UCLA) et à l'INRIA à Paris.
Mes recherches antérieures ont porté sur les domaines des équations aux dérivées partielles et du calcul scientifique; j'ai d’ailleurs apporté d'importantes contributions dans les secteurs du transport optimal numérique, des EDP géométriques et des problèmes de contrôle stochastique.
Je donne deux cours théoriques complets sur l'apprentissage automatique, couvrant des sujets tels que la théorie de l'apprentissage statistique et la théorie des noyaux.