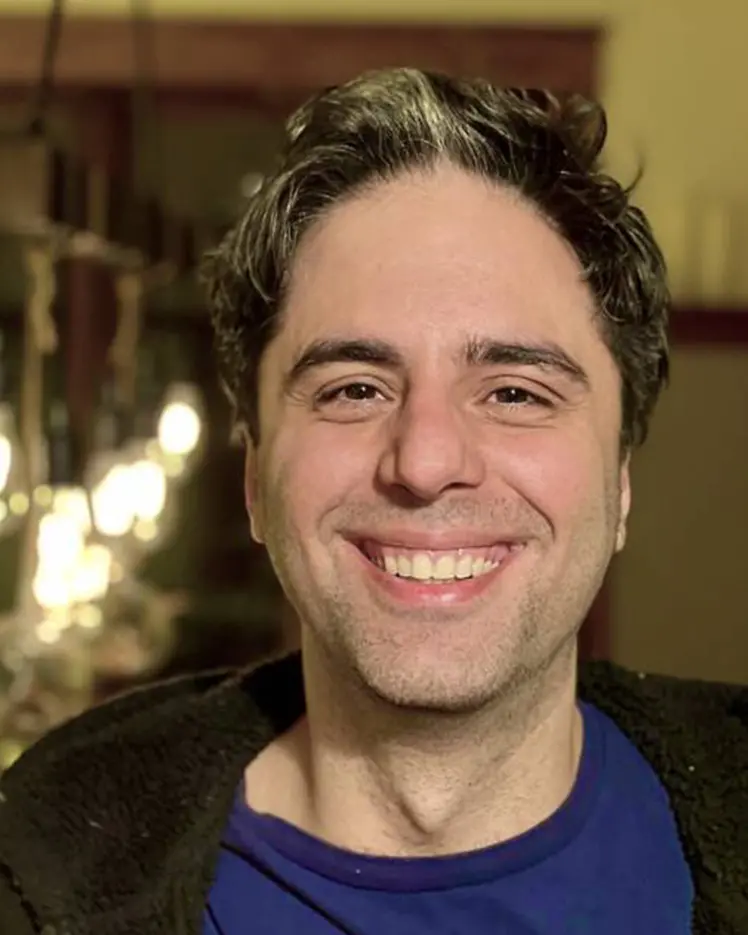
Yashar Hezaveh
Biographie
Yashar Hezaveh est membre associé de Mila – Institut québécois d'intelligence artificielle et directeur de Ciela – Institut de Montréal pour l'analyse des données astrophysiques et l'apprentissage automatique. Il est professeur adjoint au Département de physique de l'Université de Montréal, titulaire d'une chaire de recherche du Canada en analyse de données astrophysiques et apprentissage automatique, membre associé de l'Institut spatial Trottier de l'Université McGill et chercheur invité au Center for Computational Astrophysics du Flatiron Institute (New York) et au Perimeter Institute. Auparavant, il a été chercheur au Flatiron Institute (2018-2019) et boursier Hubble de la NASA à l'Université de Stanford (2013-2018).
Il est un leader mondial dans l'analyse des données astrophysiques avec l'apprentissage automatique. Ses recherches actuelles portent principalement sur l'inférence bayésienne dans l'IA (par exemple, les modèles de diffusion) et visent à faire progresser les connaissances sur la distribution de la matière noire dans les galaxies fortement lenticulaires à l'aide de données provenant de grands relevés cosmologiques. Ses recherches sont soutenues par la Schmidt Futures Foundation et la Simons Foundation.