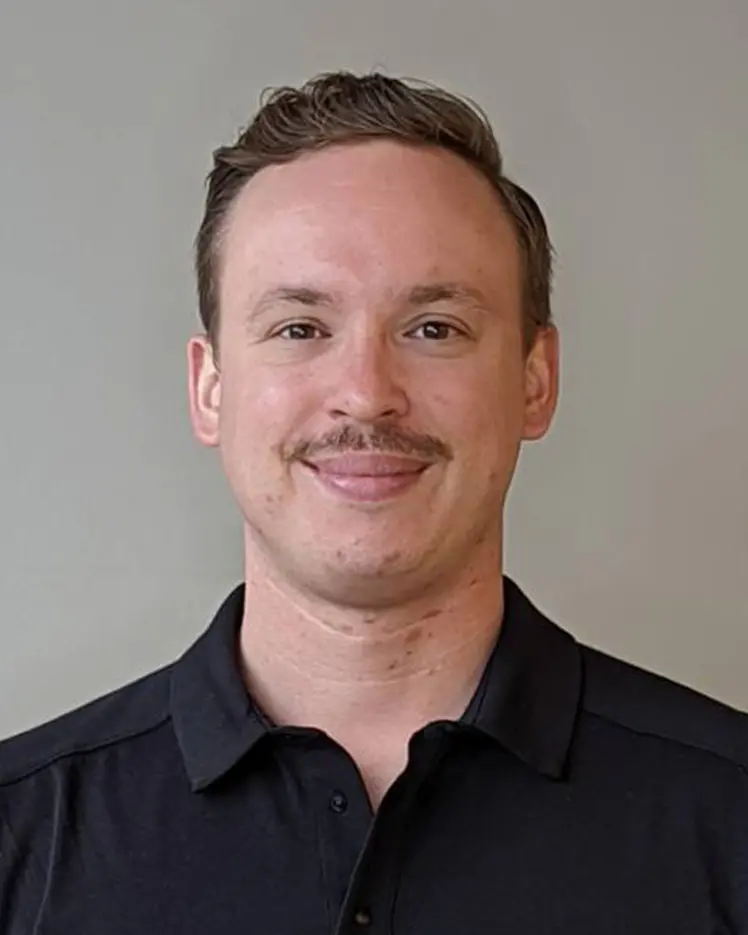
Alexandre Drouin
Biographie
Alexandre Drouin est chercheur en intelligence artificielle chez ServiceNow Research à Montréal et professeur associé au Département d’informatique et de génie logiciel de l’Université Laval. Il dirige une équipe de recherche qui explore l’utilisation de l’apprentissage automatique pour la prise de décision dans des environnements dynamiques complexes. Son intérêt de recherche principal est la prise de décision causale, dont le but est de répondre à des questions interventionnelles et contrefactuelles en tenant compte des sources d’incertitude potentielles, par exemple l’ambiguïté des relations causales sous-jacentes à un système et l’effet de variables latentes. Il s’intéresse aussi aux modèles de prédiction probabiliste pour les séries temporelles et à leur utilisation pour prédire l’effet à long terme d’actions.
Il est détenteur d’un doctorat en informatique de l’Université Laval, qu’il a reçu pour son travail sur le développement d’algorithmes d’apprentissage automatique pour la découverte de biomarqueurs en génomique et leur application au problème de résistance aux antibiotiques.