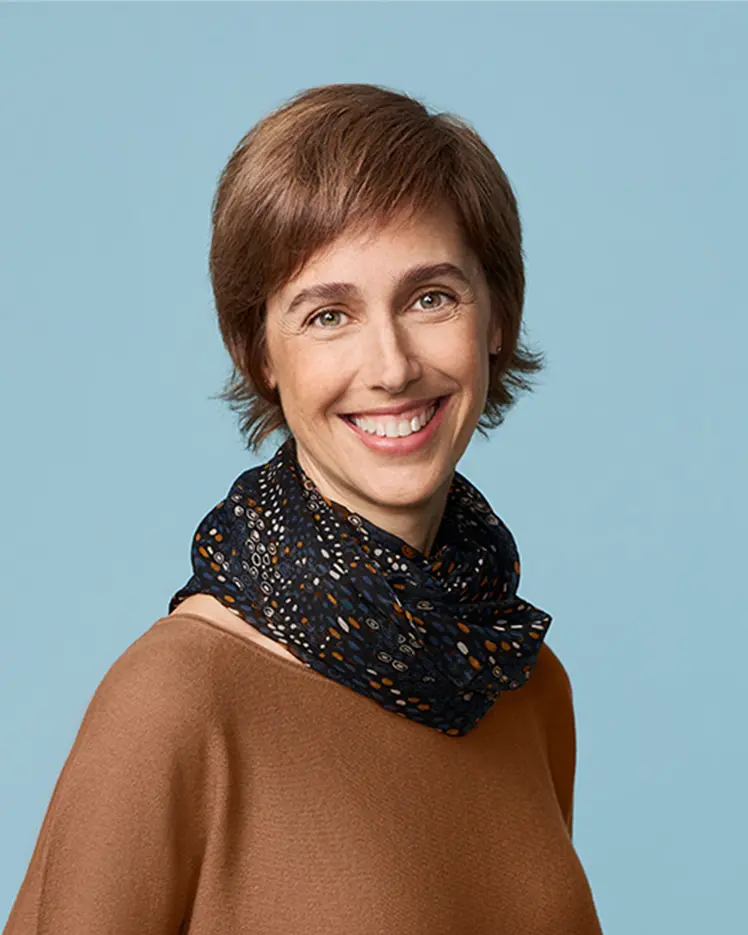
Joelle Pineau
Biographie
Joelle Pineau est professeure agrégée et titulaire d’une bourse William Dawson à l'Université McGill, où elle codirige le Laboratoire de raisonnement et d'apprentissage. Elle est membre du corps professoral de Mila – Institut québécois d’intelligence artificielle et titulaire d'une chaire en IA Canada-CIFAR. Elle est également vice-présidente de la recherche en IA chez Meta (anciennement Facebook), où elle dirige l'équipe FAIR (Fundamental AI Research). Elle détient un baccalauréat ès sciences en génie de l'Université de Waterloo et une maîtrise et un doctorat en robotique de l'Université Carnegie Mellon.
Ses recherches sont axées sur le développement de nouveaux modèles et algorithmes pour la planification et l'apprentissage dans des domaines complexes partiellement observables. Elle travaille également sur l'application de ces algorithmes à des problèmes complexes en robotique, dans les soins de santé, dans les jeux et dans les agents conversationnels. Elle est membre du comité de rédaction du Journal of Artificial Intelligence Research et du Journal of Machine Learning Research, et est actuellement présidente de l'International Machine Learning Society. Elle a été lauréate de la bourse commémorative E. W. R. Steacie du Conseil de recherches en sciences naturelles et en génie (CRSNG) 2018 et du Prix du Gouverneur général pour l'innovation 2019. Elle est membre de l'Association pour l'avancement de l'intelligence artificielle (AAAI), membre principal de l'Institut canadien de recherches avancées (CIFAR) et membre de la Société royale du Canada.