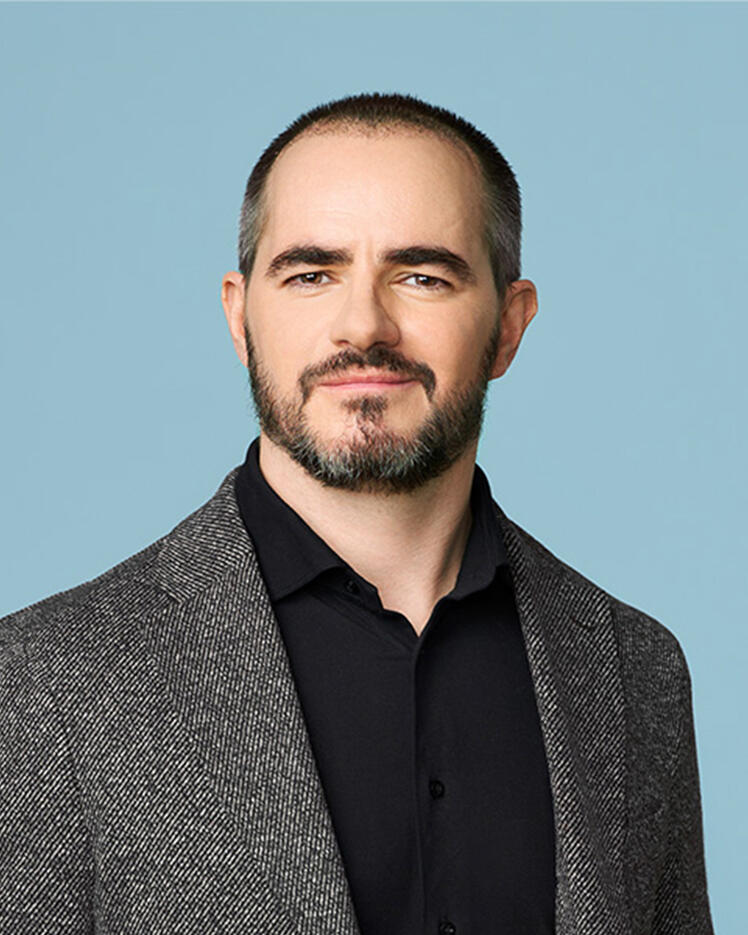
Danilo Bzdok
Biographie
Danilo Bzdok est informaticien et médecin de formation. Il possède une double formation unique en neurosciences systémiques et en algorithmes d'apprentissage automatique. Après une formation à l'Université d'Aix-la-Chapelle (RWTH) (Allemagne), à l'Université de Lausanne (Suisse) et à la Harvard Medical School (États-Unis), il a obtenu un doctorat en neurosciences du Centre de recherche de Jülich (Allemagne) et un doctorat en informatique dans le domaine des statistiques d'apprentissage automatique à l'INRIA Saclay et à NeuroSpin (Paris, France). Il est actuellement professeur agrégé à la Faculté de médecine de l'Université McGill et titulaire d’une chaire en IA Canada-CIFAR à Mila – Institut québécois d'intelligence artificielle. Son activité de recherche interdisciplinaire est centrée sur la réduction des lacunes dans la connaissance des bases cérébrales des types de pensée qui définissent l'être humain, afin de découvrir les principes clés de conception computationnelle qui sous-tendent l'intelligence humaine.