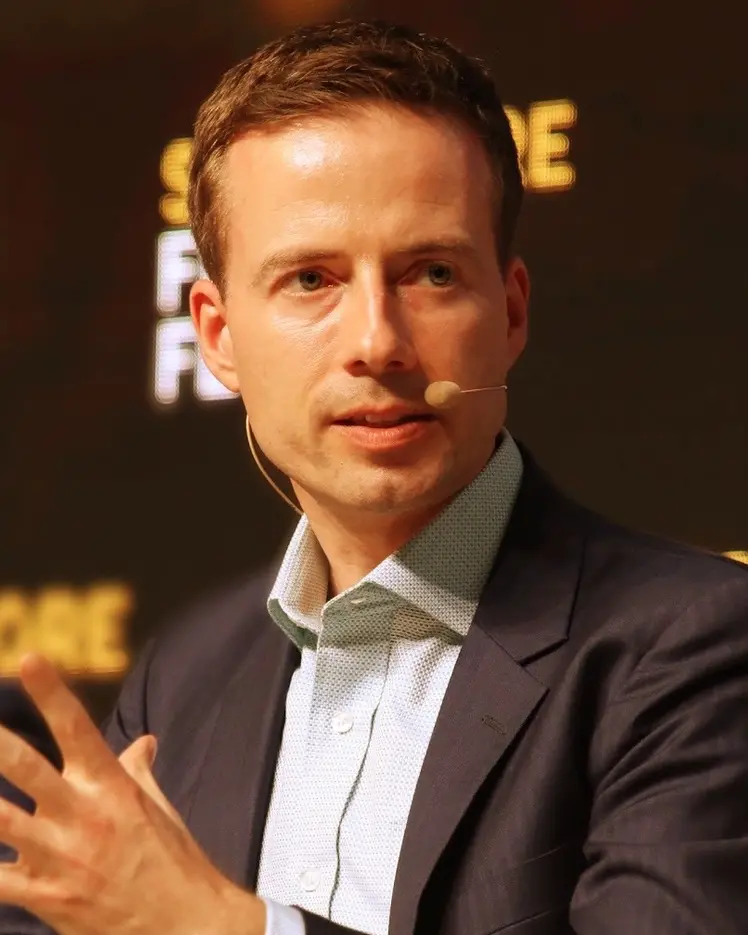
Nicolas Chapados
Biographie
Nicolas Chapados est vice-président de la recherche chez ServiceNow Inc. Il est titulaire d'un diplôme d'ingénieur de l'Université McGill et d'un doctorat en informatique de l'Université de Montréal. Conjointement avec son directeur de thèse, Yoshua Bengio, il a fondé ApSTAT Technologies en 2001. ApSTAT est une entreprise de transfert technologique visant à développer en contexte industriel des idées de pointe en apprentissage automatique, dans des domaines tels que l'évaluation des risques d'assurance, la planification de la chaîne d'approvisionnement, les prévisions commerciales, la biotechnologie et la gestion des fonds de couverture. À partir de ces travaux, il a également cofondé des entreprises dérivées : Imagia, pour détecter et quantifier précocement le cancer grâce à l'analyse d'images médicales par l'IA; Element AI (acquise par ServiceNow en janvier 2021); et Chapados Couture Capital, un gestionnaire d'actifs quantitatifs. Ses intérêts de recherche comprennent la modélisation de séries temporelles, le traitement du langage naturel et la prise de décisions. Il est titulaire du titre d'analyste financier agréé (CFA).