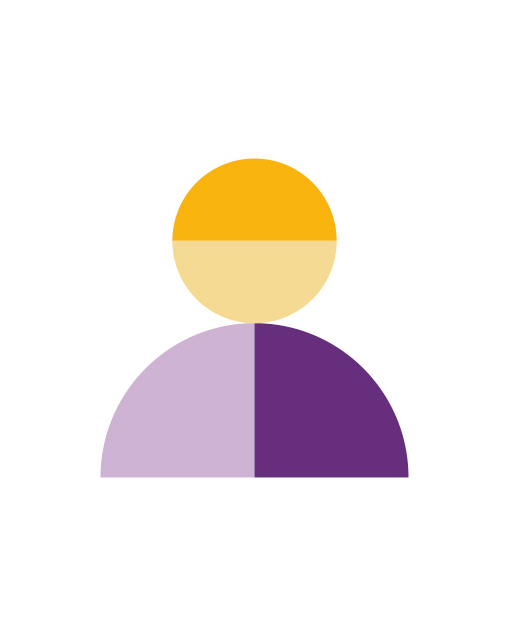
Laurence Perreault-Levasseur
Biographie
Laurence Perreault-Levasseur est titulaire de la Chaire de recherche du Canada en cosmologie computationnelle et en intelligence artificielle. Elle est professeure adjointe à l'Université de Montréal et membre associée de Mila – Institut québécois d’intelligence artificielle, où elle mène des recherches sur le développement et l'application de méthodes d'apprentissage automatique à la cosmologie. Elle est également chercheuse invitée au Flatiron Institute, à New York. Auparavant, elle a été chargée de recherche au Center for Computational Astrophysics du Flatiron Institute et boursière postdoctorale du KIPAC à l'Université de Stanford. Laurence Perreault-Levasseur a obtenu un doctorat de l'Université de Cambridge, où elle a travaillé sur les applications des méthodes de la théorie des champs effectifs ouverts au formalisme de l'inflation. Elle est titulaire d'une licence et d'une maîtrise en sciences de l'Université McGill.