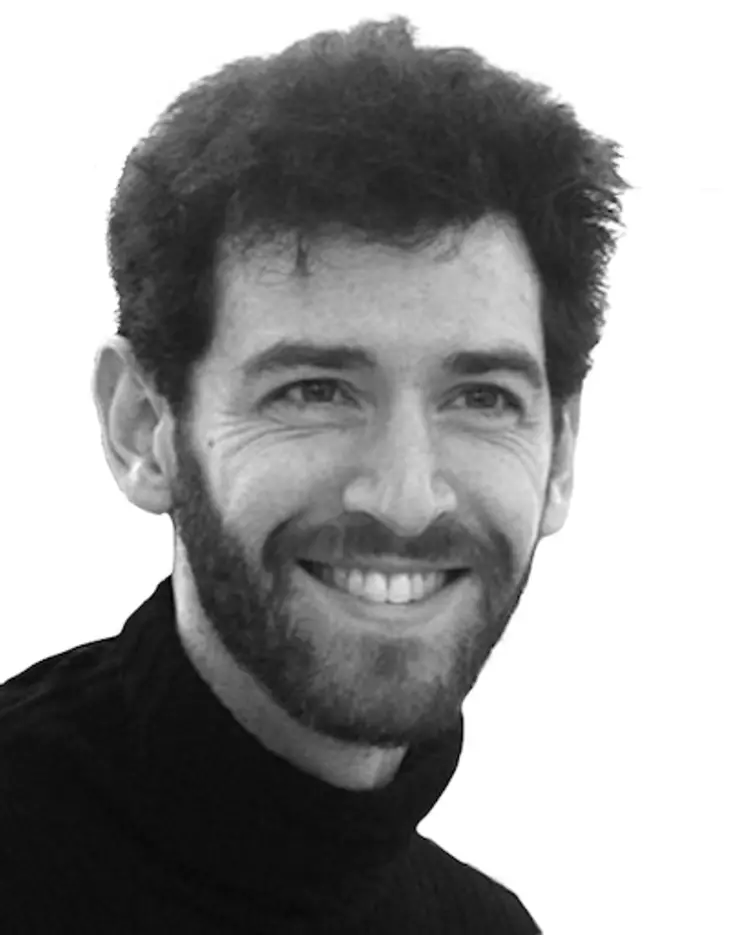
Julien Cohen-Adad
Biographie
Julien Cohen-Adad est professeur à Polytechnique Montréal et directeur associé de l'Unité de neuro-imagerie fonctionnelle de l'Université de Montréal. Il est également titulaire de la Chaire de recherche du Canada en imagerie par résonance magnétique quantitative. Ses recherches portent sur l'avancement des méthodes de neuro-imagerie avec l'aide de l'IA. Voici quelques exemples de ses projets :
- Formation multimodale pour les tâches d'imagerie médicale (segmentation des pathologies, diagnostic, etc.);
- Ajout d'un a priori issu de la physique de l'IRM pour améliorer la généralisation des modèles;
- Incorporation de mesures d'incertitude pour traiter la variabilité interévaluateurs;
- Stratégies d'apprentissage continu lorsque le partage des données est restreint;
- Introduction des méthodes d'IA dans la routine de la radiologie clinique par l’intermédiaire de solutions logicielles conviviales.
Le professeur Cohen-Adad dirige également de nombreux projets de logiciels libres qui profitent à la communauté scientifique et clinique. Plus de détails sur https://neuro.polymtl.ca/software.html.
En résumé, Julien aime : l'IRM avec des aimants puissants, la neuro-imagerie, la programmation et la science ouverte!