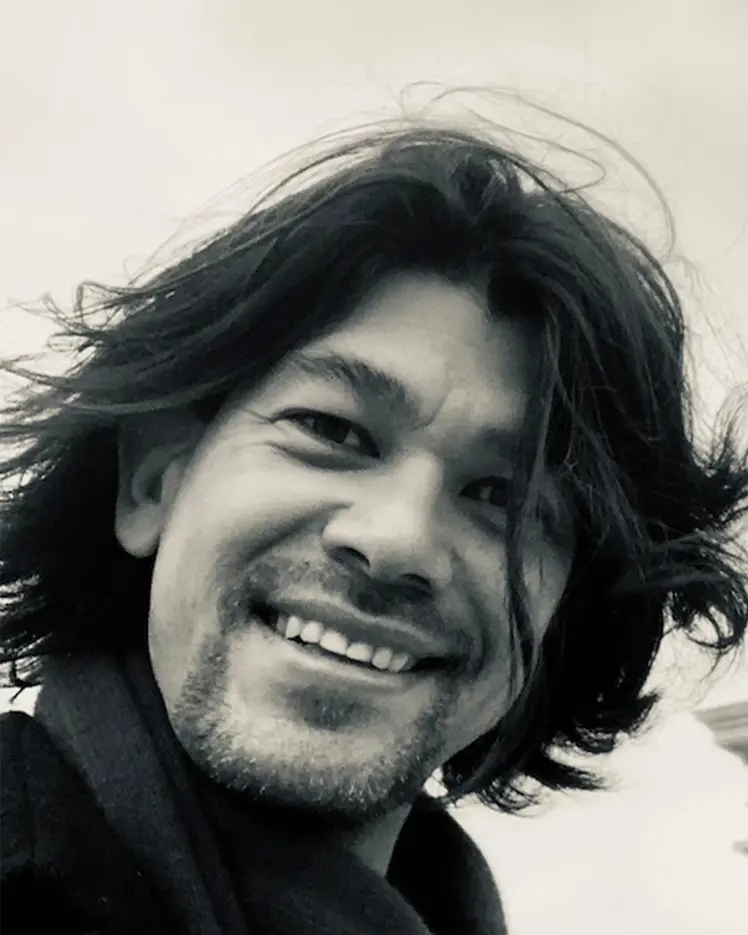
Hervé Lombaert
Biographie
Hervé Lombaert est professeur agrégé à Montréal, où il est titulaire d'une Chaire de recherche du Canada sur l’analyse de formes en imagerie médicale. Ses recherches portent sur les statistiques et l'analyse des formes dans le contexte de l'apprentissage automatique et de l'imagerie médicale. Ses travaux sur l'analyse de graphes ont eu une influence sur plusieurs applications en imagerie médicale, depuis les premières segmentations d'images par graph cuts jusqu'aux récentes analyses de surface par théorie de graphes spectraux. Hervé Lombaert est l'auteur de plus de 70 articles. Il détient 5 brevets et a remporté plusieurs prix, dont le prix Erbsmann de l’Information Processing in Medical Imaging (IPMI). Ses étudiant·e·s ont également reçu des prix de la meilleure thèse pour des publications marquantes en imagerie médicale. Il est éditeur associé de Medical Image Analysis. Il a aussi eu la chance de travailler dans plusieurs centres, dont Inria Sophia-Antipolis (France), Microsoft Research (Cambridge, Royaume-Uni), Siemens Corporate Research (Princeton, NJ, É-U), l’Université McGill et Polytechnique Montréal.