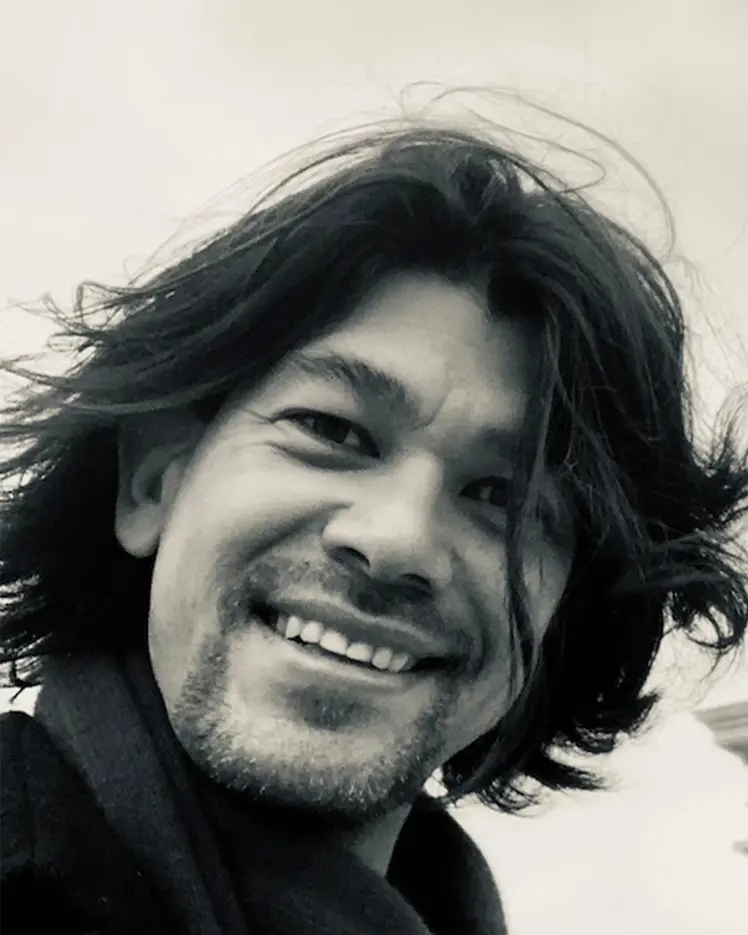
Hervé Lombaert
Biography
Hervé Lombaert is an associate professor in the Computer Engineering Department at Polytechnique Montréal, and the Canada Research Chair in Shape Analysis in Medical Imaging. His research focuses on the statistics and analysis of shapes in the context of machine learning and medical imaging. His work on graph analysis has impacted several applications in medical imaging, from early image segmentation with graph-cuts to recent surface analysis with spectral graph theory.
Lombaert has authored over seventy papers, holds five patents and has earned several awards, including the IPMI Erbsmann Prize. His students have also received best thesis awards with impactful publications in medical image computing. He is on the editorial board of Medical Image Analysis. He has also worked in a number of other research centres, including the INRIA Sophia-Antipolis (France), Microsoft Research (Cambridge, U.K.), Siemens Corporate Research (Princeton, NJ) and McGill University.