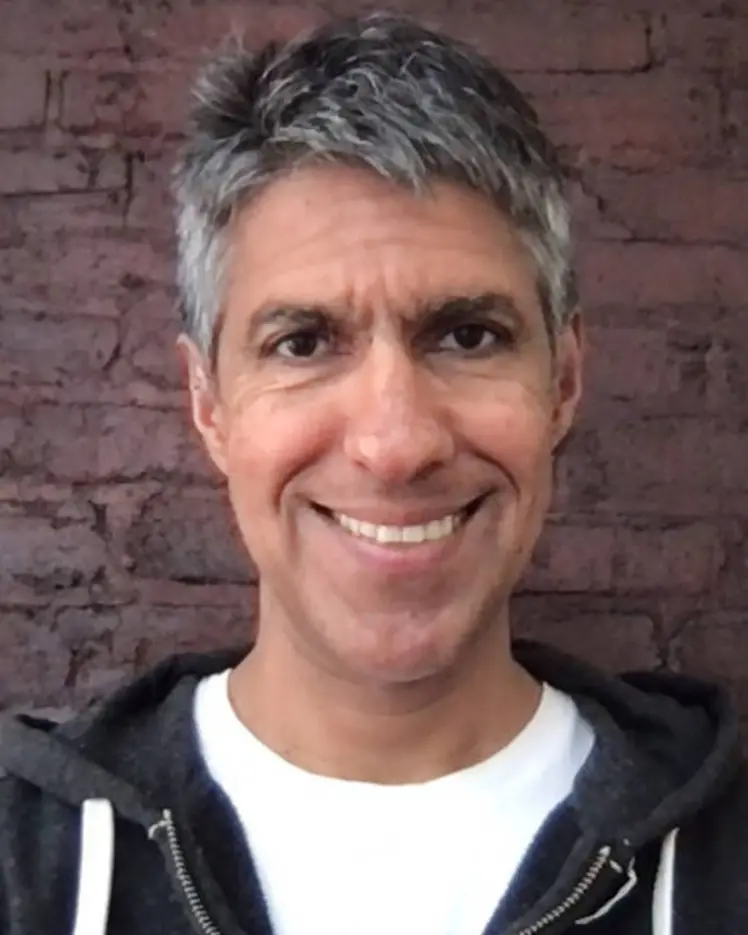
Kaleem Siddiqi
Biography
Kaleem Siddiqi is a professor of computer science at McGill University and a member of McGill’s Centre for Intelligent Machines. He is also an associate academic member of Mila – Quebec Artificial Intelligence Institute, McGill’s Department of Mathematics and Statistics, and the Goodman Centre for Cancer Research at McGill. He holds an FRQS Dual Chair in Artificial Intelligence and Health with Keith Murai. Siddiqi’s research interests lie in computer vision, biological image analysis, neuroscience, visual perception and robotics. He is field chief editor for Frontiers in Computer Science and has served as an associate editor of IEEE Transactions on Pattern Analysis and Machine Intelligence, Pattern Recognition and Frontiers in ICT. He is co-author with Steve Pizer of the book Medial Representations: Mathematics, Algorithms and Applications (Springer, 2008).