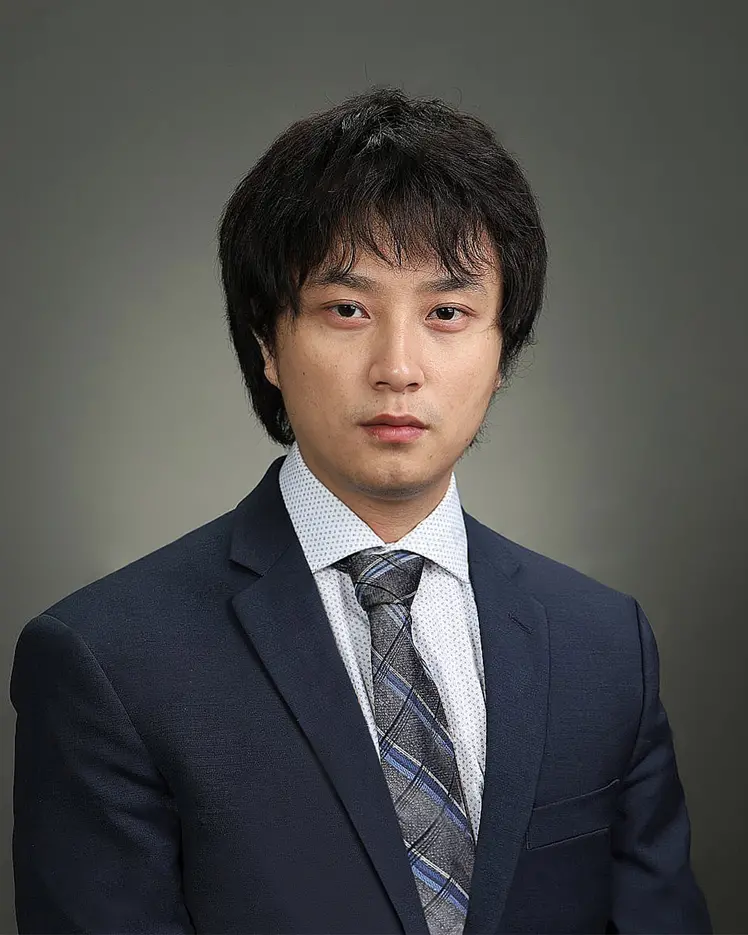
Bang Liu
Biographie
Bang Liu est professeur adjoint au Département d'informatique et de recherche opérationnelle (DIRO) de l'Université de Montréal. Il est membre du Laboratoire de recherche appliquée en linguistique informatique (RALI) du DIRO, membre associé de Mila – Institut québécois d'intelligence artificielle, et titulaire d'une chaire en IA Canada-CIFAR.
Il a obtenu un baccalauréat en ingénierie de l'Université des sciences et technologies de Chine (USTC) en 2013, ainsi qu’une maîtrise ès sciences et un doctorat de l'Université de l'Alberta en 2015 et en 2020, respectivement. Ses recherches portent principalement sur le traitement du langage naturel, l'apprentissage multimodal et incarné, la théorie et les techniques de l'intelligence artificielle (par exemple, la compréhension et l'amélioration de grands modèles de langage) et l'intelligence artificielle pour la science (par exemple, la santé, la science des matériaux et la radiologie).