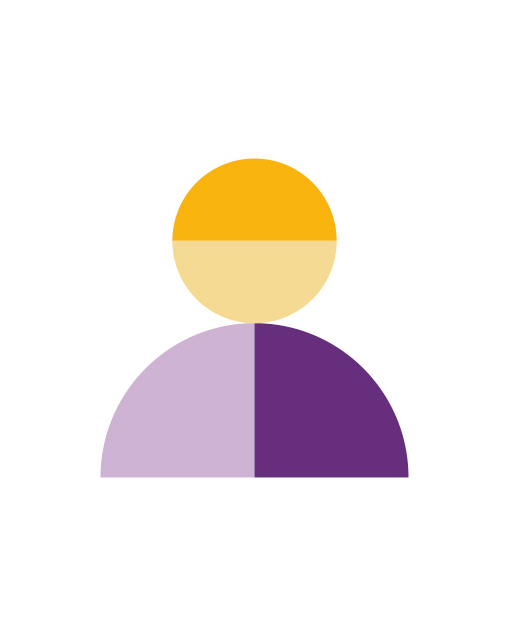
Marc Gendron-Bellemare
Biographie
J'occupe actuellement le poste de directeur scientifique à Reliant AI. Je suis également professeur adjoint à l'École d'informatique de l'Université McGill et professeur adjoint au Département d'informatique et de recherche opérationnelle (DIRO) de l'Université de Montréal.
Précédemment, j'ai travaillé à Google Brain à Montréal, où je me concentrais sur l'apprentissage par renforcement. De 2013 à 2017, j'ai travaillé chez DeepMind au Royaume-Uni. J'ai obtenu un doctorat de l'Université de l'Alberta en travaillant avec Michael Bowling et Joel Veness.
Ma recherche se situe au carrefour de l'apprentissage par renforcement et de la prédiction probabiliste. Je m'intéresse aussi à l'apprentissage profond, à la modélisation générative, à l'apprentissage en ligne et à la théorie de l'information.