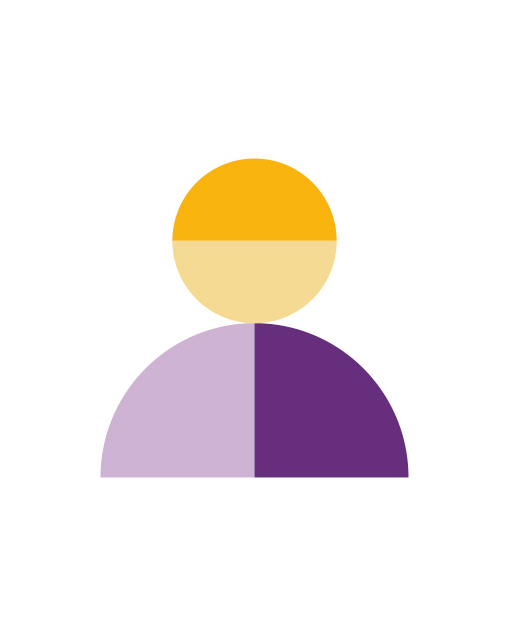
Marc Gendron-Bellemare
Biography
I am Chief Scientific Officer at Reliant AI, an adjunct professor at the School of Computer and Science at McGill University, and an adjunct professor at the Department of Computer Science and Operations Research (DIRO) at Université de Montréal.
Previously, I was a research scientist at Google Brain in Montréal, where my research focused on reinforcement learning effort. From 2013 to 2017, I worked at DeepMind in the U.K. I received my PhD from the University of Alberta under the supervision of Michael Bowling and Joel Veness.
My research lies at the intersection of reinforcement learning and probabilistic prediction. I am also interested in deep learning, generative modelling, online learning and information theory.