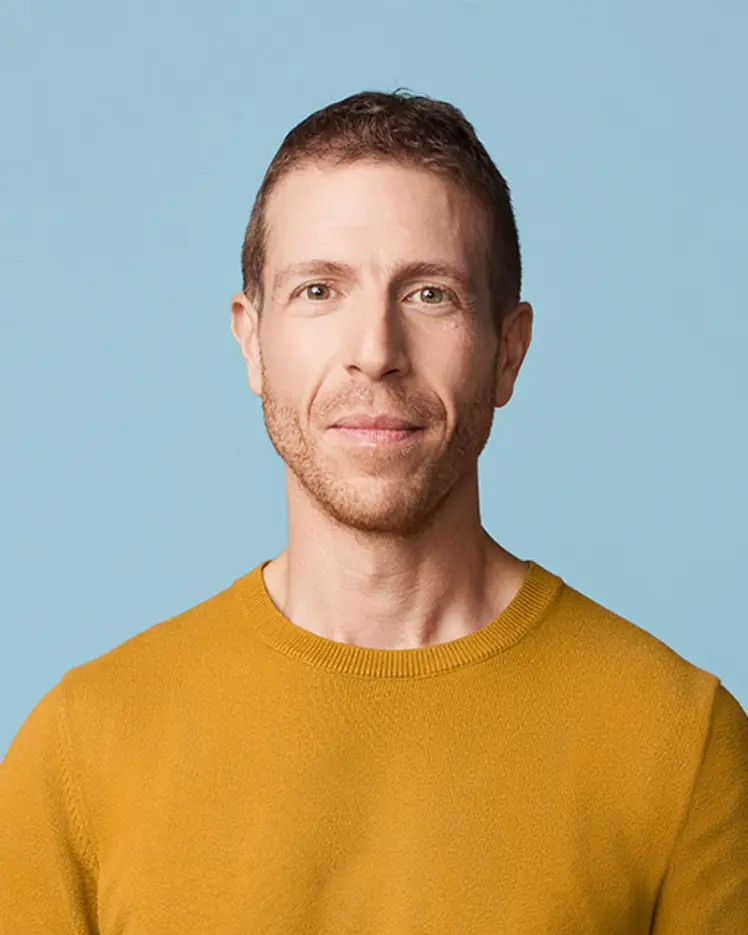
Derek Nowrouzezahrai
Biographie
Derek Nowrouzezahrai est professeur titulaire à l'Université McGill, directeur du Centre sur les machines intelligentes et codirecteur du Laboratoire de graphisme et d’imagerie de McGill (MGIL), ainsi que titulaire d’une chaire en IA Canada-CIFAR et de la chaire Ubisoft-Mila de mise à l'échelle des univers de jeux grâce à une IA responsable.
Ses recherches portent sur la simulation de divers phénomènes physiques - tels que la dynamique des objets en mouvement et l'éclairage pour la synthèse d'images réalistes - avec des applications dans les domaines de la réalité virtuelle, des jeux vidéo, de la simulation fluide et contrôlée, de la fabrication numérique, de l'optique augmentée par le calcul et du traitement de la géométrie. En outre, Derek s'intéresse au développement de simulateurs dérivables de ces systèmes dynamiques et à leurs applications aux problèmes inverses en robotique et dans le domaine de la vision.
Son travail repose sur le développement de méthodes Monte Carlo à haute performance et efficaces en matière d'échantillonnage (chaîne de Markov), de statistiques d'ordre élevé et de méthodes de calcul pour les problèmes d'intégration multidimensionnelle complexes, de simulateurs dérivables basés sur la physique et de méthodes numériques pour les systèmes dynamiques, ainsi que sur l'application de l'apprentissage automatique aux médias 3D, visuels et interactifs.