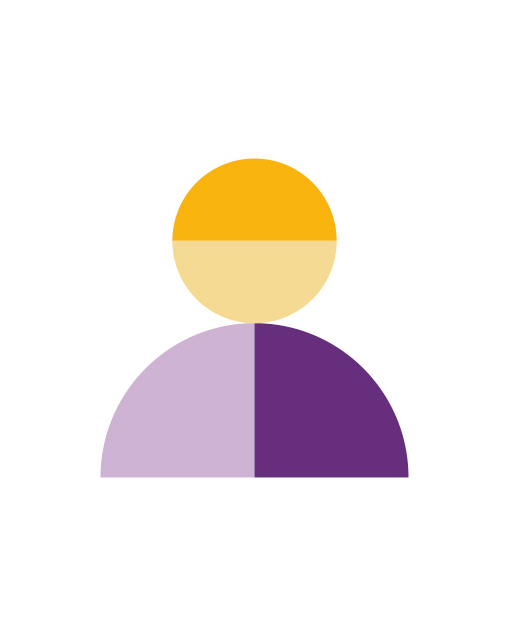
Pouya Bashivan
Biographie
Pouya Bashivan est professeur adjoint au Département de physiologie et membre du programme intégré en neurosciences de l'Université McGill, ainsi que membre associé de Mila – Institut québécois d'intelligence artificielle. Avant de se joindre à l'Université McGill, il a été chercheur postdoctoral à Mila, travaillant avec Irina Rish et Blake Richards. Auparavant, il a été chercheur postdoctoral au Département des sciences du cerveau et de la cognition et à l'Institut McGovern pour la recherche sur le cerveau du Massachusetts Institute of Technology (MIT), où il a travaillé avec le professeur James DiCarlo. Il a obtenu un doctorat en génie informatique de l'Université de Memphis en 2016, après avoir obtenu une licence et une maîtrise en ingénierie électrique et de contrôle de l'Université KNT (Téhéran, Iran).
L'objectif de la recherche menée à son laboratoire est de développer des modèles de réseaux neuronaux qui exploitent la mémoire pour résoudre des tâches complexes. Alors que nous nous appuyons souvent sur des mesures de performance des tâches pour trouver des modèles de réseaux neuronaux et des algorithmes d'apprentissage améliorés, nous utilisons également des mesures neuronales et comportementales provenant de cerveaux d’humains et d'autres animaux pour évaluer la similitude de ces modèles avec des cerveaux biologiquement évolués. Nous pensons que ces contraintes supplémentaires pourraient accélérer les progrès vers l'ingénierie d'un agent artificiellement intelligent de niveau humain.