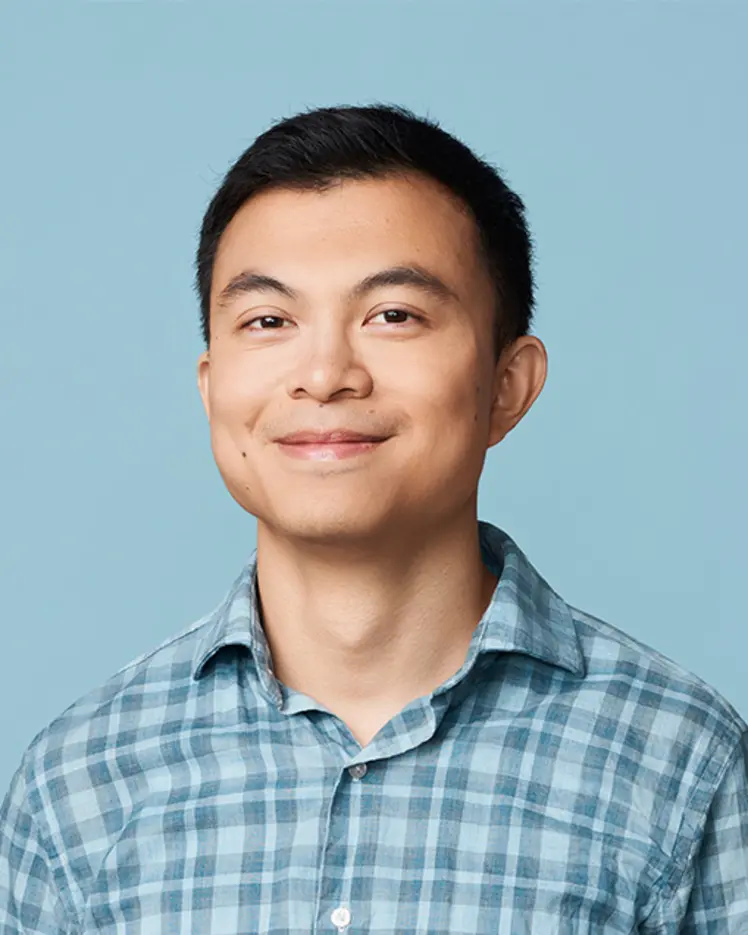
Jackie Cheung
Biographie
Je suis professeur agrégé à l'École d’informatique de l’Université McGill et chercheur consultant à Microsoft Research.
Mon groupe mène des recherches sur le traitement du langage naturel (NLP), un domaine de l'intelligence artificielle qui implique la construction de modèles informatiques de langages humains tels que l'anglais ou le français. Le but de nos recherches est de développer des méthodes informatiques de compréhension du texte et de la parole, afin de générer un langage fluide et adapté au contexte.
Dans notre laboratoire, nous étudions des techniques statistiques d’apprentissage automatique pour analyser et faire des prédictions sur la langue. Plusieurs projets en cours incluent la synthèse de fiction, l'extraction d'événements à partir d’un texte et l'adaptation de la langue à différents genres.