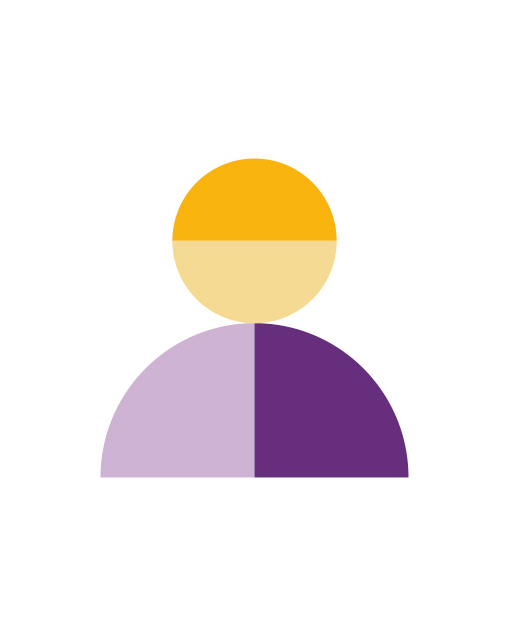
Eugene Belilovsky
Biographie
Eugene Belilovsky est professeur adjoint au Département d'informatique et de génie logiciel de l'Université Concordia. Il est également membre associé de Mila – Institut québécois d’intelligence artificielle et professeur adjoint à l'Université de Montréal. Ses travaux se concentrent sur la vision par ordinateur et l'apprentissage profond. Ses intérêts de recherche actuels comprennent l'apprentissage continu, l'apprentissage à partir de peu de données (few-shot learning) et leurs applications au carrefour de la vision par ordinateur et du traitement du langage.