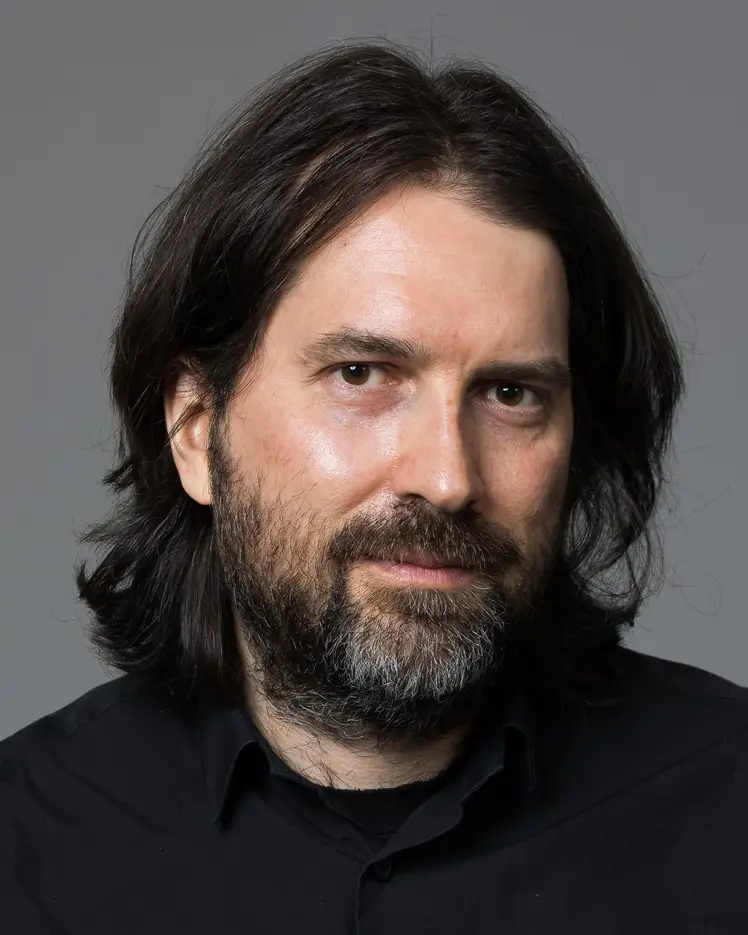
Christian Gagné
Biographie
Christian Gagné est professeur au Département de génie électrique et de génie informatique de l’Université Laval depuis 2008, et dirige l’Institut intelligence et données (IID). Il détient une chaire en IA Canada-CIFAR et est membre associé à Mila – Institut québécois d’intelligence artificielle. Il est également membre du Laboratoire de vision et systèmes numériques (LVSN), une composante du Centre de recherche en robotique, vision et intelligence machine (CeRVIM) ainsi que du Centre de recherche en données massives (CRDM) de l’Université Laval. Il fait partie des regroupements stratégiques REPARTI et UNIQUE du Fonds de recherche du Québec – Nature et technologies (FRQNT), du centre VITAM du Fonds de recherche du Québec – Santé (FRQS) et de l’Observatoire international sur les impacts sociétaux de l’IA et du numérique (OBVIA).
Ses intérêts de recherche portent sur l’élaboration de méthodes pour l’apprentissage automatique et l’optimisation stochastique. En particulier, il se consacre aux réseaux de neurones profonds, à l’apprentissage et au transfert de représentations, au méta-apprentissage ainsi qu’à l’apprentissage multitâche. Il s’intéresse également aux approches d’optimisation basées sur des modèles probabilistes ainsi qu’aux algorithmes évolutionnaires, entre autres pour l’optimisation boîte noire et la programmation automatique. Une part importante de ses travaux porte également sur la mise en pratique de ces techniques dans des domaines comme la vision numérique, la microscopie, la santé, l’énergie et les transports.