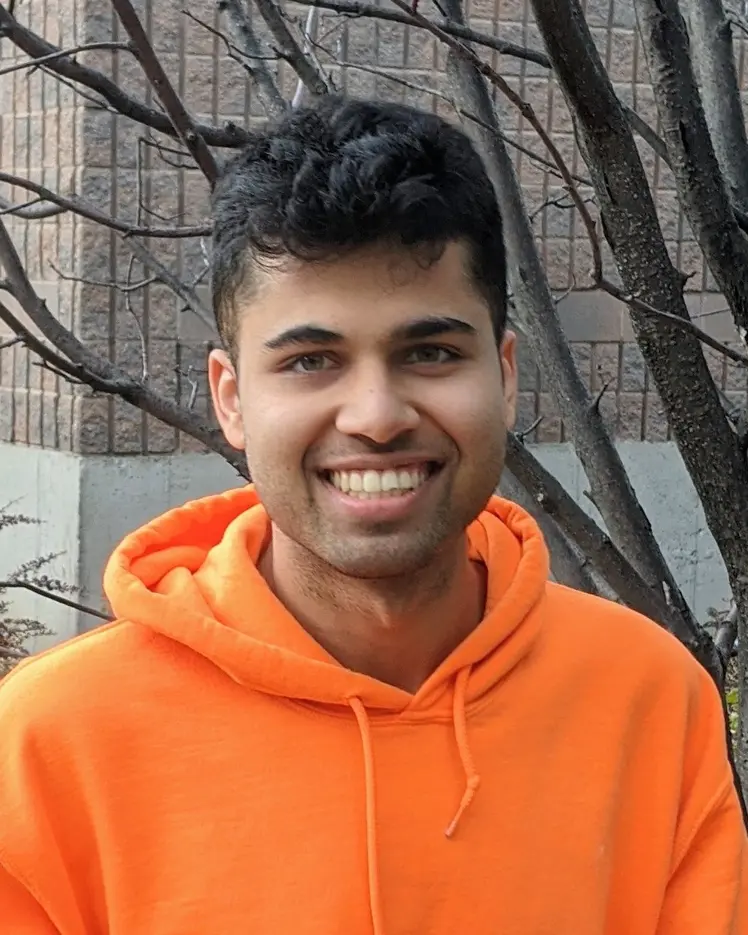
Rishabh Agarwal
Biographie
Je suis chercheur dans l'équipe DeepMind de Google à Montréal, professeur adjoint à l'Université McGill et membre industriel associé à Mila - Institut québécois d'intelligence artificielle. J'ai réalisé mon doctorat au sein de Mila sous la supervision d'Aaron Courville et Marc Bellemare. Avant cela, j'ai eu l'opportunité de travailler pendant un an avec l'équipe de Geoffrey Hinton chez Google Brain, à Toronto. J'ai obtenu mon diplôme en informatique et en ingénierie à l'IIT Bombay.
Mes recherches se concentrent sur les modèles de langage et l'apprentissage par renforcement profond (RL). J'ai eu l'honneur de recevoir un prix pour un article exceptionnel présenté à NeurIPS.