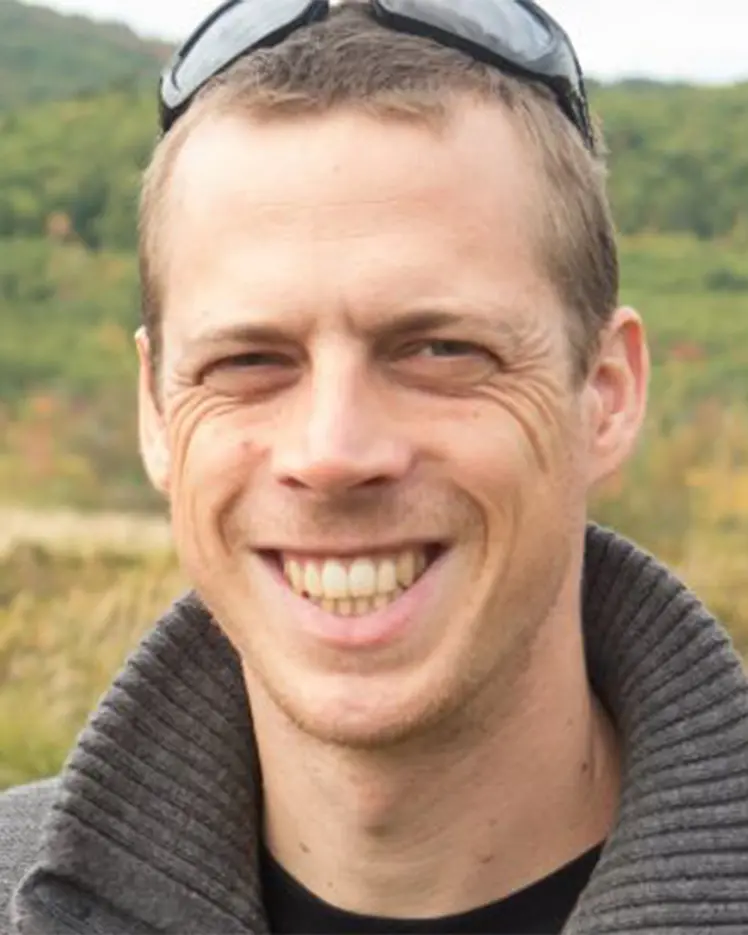
Nicolas Le Roux
Membre industriel principal
Chaire en IA Canada-CIFAR
Professeur associé, McGill University, École d'informatique
Professeur associé, Université de Montréal, Département d'informatique et de recherche opérationnelle
Chercheur scientifique, Microsoft Research
Biographie
Je suis un chercheur universitaire spécialisé dans l'apprentissage automatique, la vision par ordinateur, les réseaux de neurones, l'apprentissage en profondeur, l'optimisation, l'apprentissage à grande échelle et la modélisation statistique en général.