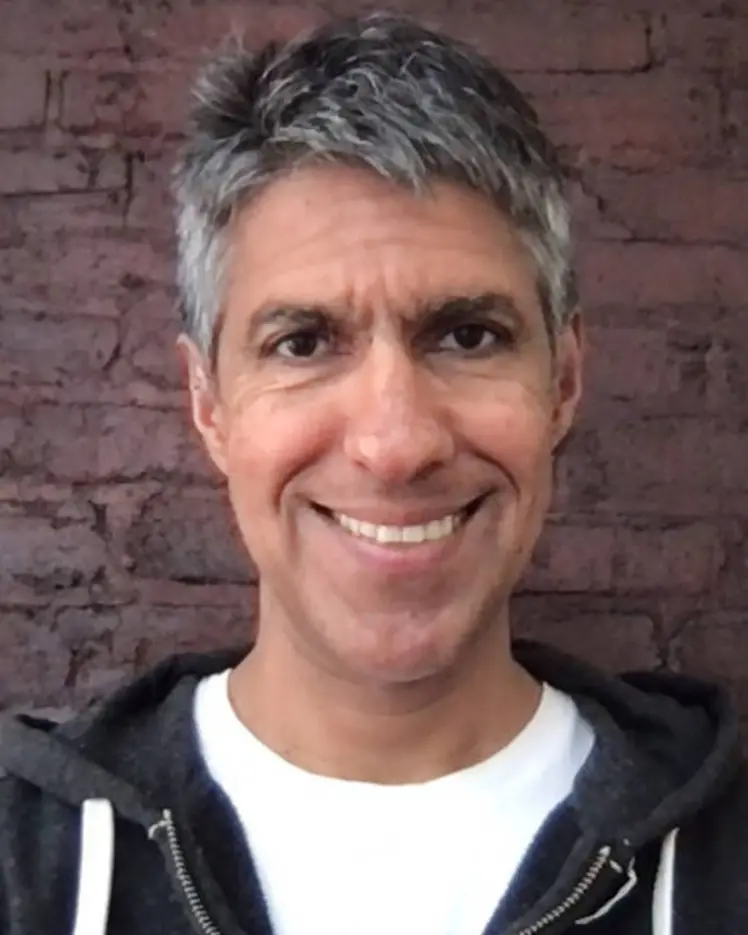
Kaleem Siddiqi
Biographie
Kaleem Siddiqi est professeur d'informatique à l'Université McGill et membre du Centre sur les machines intelligentes de McGill. Il est membre académique associé de Mila – Institut québécois d’intelligence artificielle, du Département de mathématiques et de statistiques de McGill et du Centre de recherche sur le cancer Goodman de McGill. Il est titulaire d'une Chaire de recherche double en intelligence artificielle en santé / santé numérique et sciences de la vie du Fonds de recherche du Québec - Santé (FRQS) avec Keith Murai. Ses recherches portent sur la vision par ordinateur, l'analyse d'images biologiques, les neurosciences, la perception visuelle et la robotique. Il est rédacteur en chef du journal Frontiers in Computer Science et a été rédacteur en chef adjoint des IEEE Transactions on Pattern Analysis and Machine Intelligence (PAMI), de Pattern Recognition et de Frontiers in ICT. Il est coauteur, avec Steve Pizer, du livre Medial Representations: Mathematics, Algorithms and Applications (Springer, 2008).