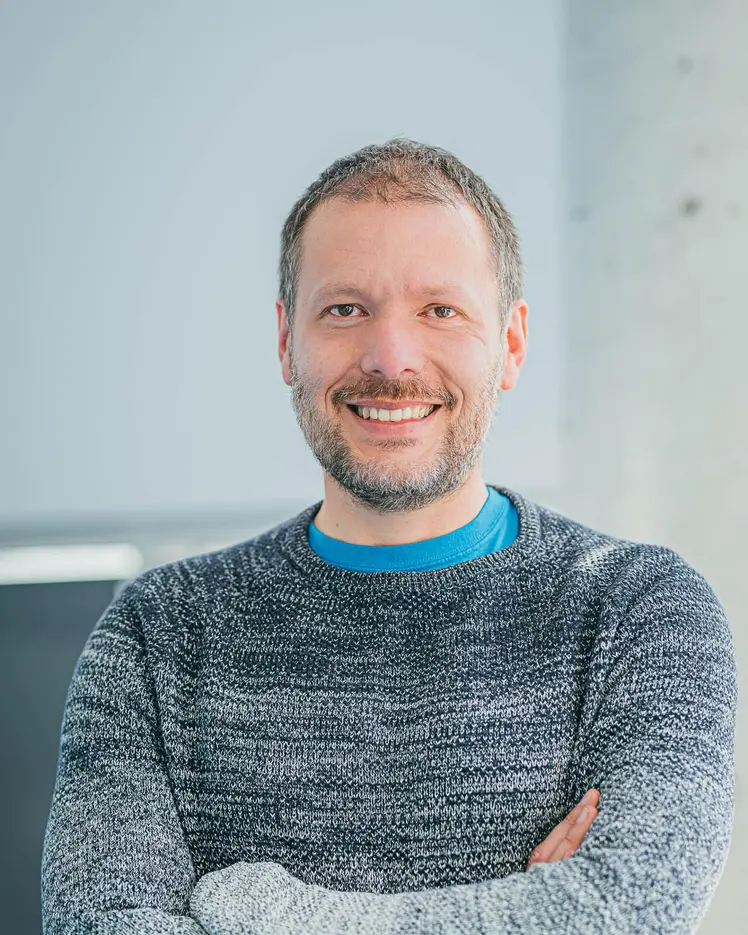
Giovanni Beltrame
Biographie
Giovanni Beltrame a obtenu un doctorat en génie informatique du Politecnico di Milano en 2006, après quoi il a travaillé comme ingénieur en microélectronique à l'Agence spatiale européenne (ESA) sur un certain nombre de projets, allant des systèmes tolérants aux radiations à la conception assistée par ordinateur. En 2010, il s'est installé à Montréal. Il est actuellement professeur au Département de génie informatique et logiciel de Polytechnique Montréal. Il dirige notamment le laboratoire MIST, qui se consacre aux technologies spatiales, où plus de 25 étudiant·e·s et postdoctorant·e·s sont sous sa supervision. Il a réalisé plusieurs projets en collaboration avec l'industrie et les agences gouvernementales dans les domaines de la robotique, de l'intervention en cas de catastrophe et de l'exploration spatiale. Avec son équipe, il a participé à plusieurs missions sur le terrain avec l'ESA, l'Agence spatiale canadienne (ASC) et la NASA (BRAILLE, PANAGAEA-X et IGLUNA, entre autres). Ses recherches portent sur la modélisation et la conception de systèmes embarqués, l'intelligence artificielle et la robotique, sujets sur lesquels il a publié plusieurs articles dans des revues et des conférences de premier plan.