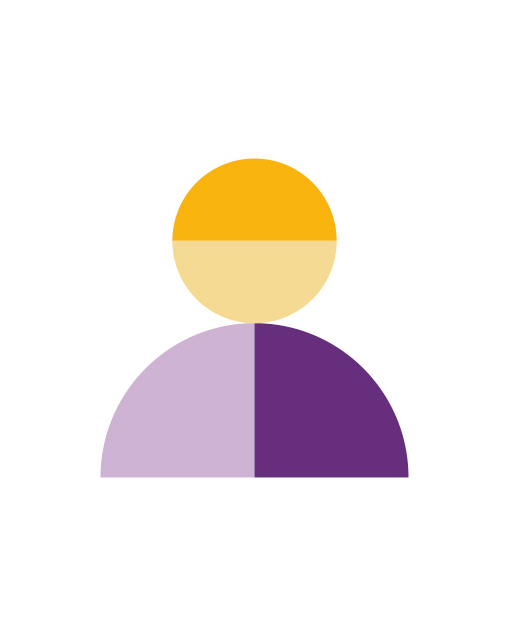
Alessandro Sordoni
Biographie
Je suis chercheur principal à Microsoft Research Montréal. J'ai obtenu un doctorat de l'Université de Montréal sous la direction de Jian-Yun Nie, en étudiant comment représenter efficacement les documents et les requêtes pour la recherche d'information. Présentement, je m’intéresse à l'étude de l'efficacité de l'apprentissage et de la généralisation systématique dans les grands modèles actuels d'apprentissage profond. Mes intérêts s'étendent à l'apprentissage non supervisé et à l'apprentissage à petite échelle, en particulier dans le domaine du langage naturel.