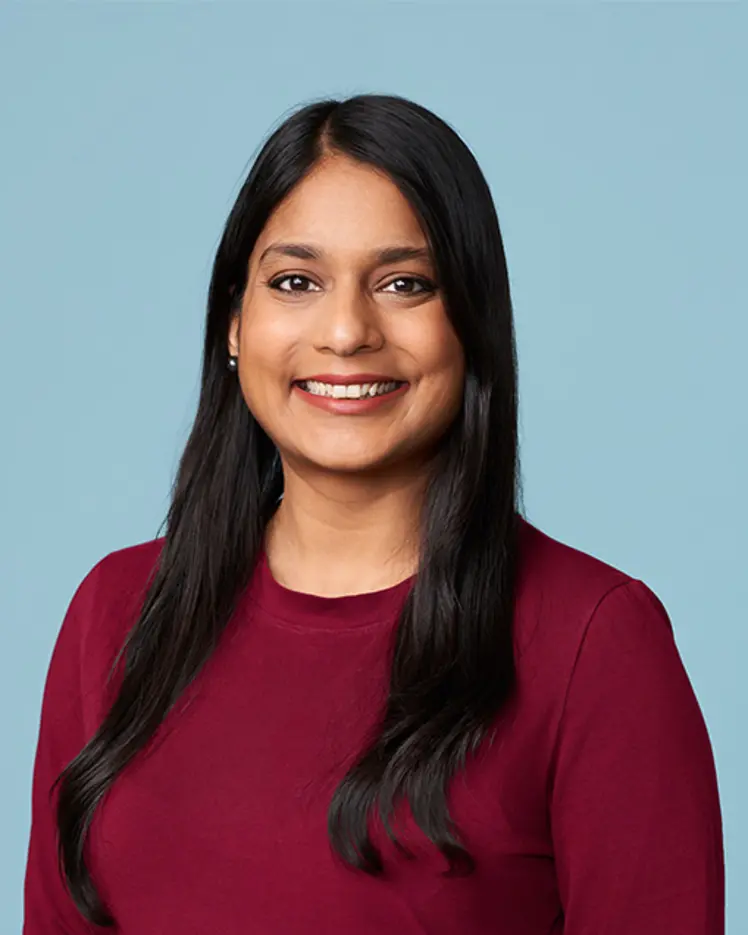
Aishwarya Agrawal
Biographie
Aishwarya Agrawal est professeure adjointe au Département d'informatique et de recherche opérationnelle (DIRO) de l'Université de Montréal. Elle est également titulaire d'une chaire en IA Canada-CIFAR et membre académique principale de Mila – Institut québécois d’intelligence artificielle.
Elle passe également un jour par semaine chez DeepMind en tant que chercheuse scientifique; d'août 2019 à décembre 2020, elle y a été chercheuse scientifique à plein temps. Détentrice d’un baccalauréat en génie électrique avec une mineure en informatique, Aishwarya a obtenu en août 2019 un doctorat de Georgia Tech, en travaillant avec Dhruv Batra et Devi Parikh. Ses intérêts de recherche se situent à l'intersection des sous-disciplines suivantes de l'IA : vision par ordinateur, apprentissage profond et traitement du langage naturel, avec un accent sur le développement de systèmes d'IA capables de « voir » (c'est-à-dire de comprendre le contenu d'une image : qui, quoi, où, qui fait quoi ?) et de « parler » (c'est-à-dire de communiquer cette compréhension aux humains en langage naturel libre).
Elle a reçu plusieurs prix et bourses, dont le prix des chaires en IA Canada-CIFAR, le prix de la meilleure thèse de doctorat Sigma Xi 2020 et le prix de la dissertation 2020 du College of Computing de Georgia Tech, la bourse Google 2019 et la bourse Facebook 2019-2020 (toutes deux refusées en raison de l'obtention du diplôme), ainsi que la bourse d’études supérieures NVIDIA 2018-2019. Aishwarya a été l'une des deux finalistes du prix de la meilleure thèse 2019 de l'AAAI / ACM SIGAI. Elle a également été sélectionnée pour les Rising Stars in EECS 2018.