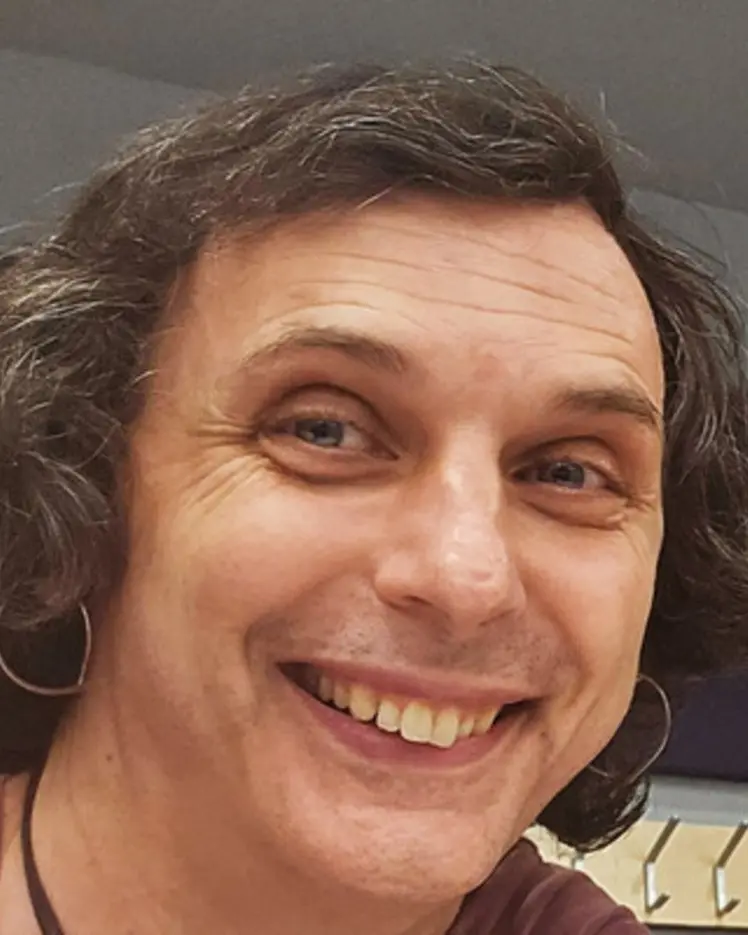
Lune Bellec
Biographie
Je suis professeur agrégé au Département de psychologie de l'Université de Montréal et chercheur principal du Laboratoire de simulation cérébrale et d'exploration (SIMEXP) de l'Institut universitaire de gériatrie de Montréal (CRIUGM). Je me suis joint récemment à Mila – Institut québécois d'intelligence artificielle en tant que membre affilié et je supervise des étudiant·e·s en informatique (neurosciences computationnelles cognitives) au Département d’informatique et de recherche opérationnelle (DIRO) de l'Université de Montréal. Mes travaux de recherche consistent principalement à entraîner des réseaux neuronaux artificiels afin de reproduire conjointement l'activité cérébrale et le comportement individuel humain. Pour atteindre cet objectif, je dirige un effort intensif de collecte de données individuelles en neuro-imagerie (IRM fonctionnelle, magnétoencéphalographie) : le projet Courtois sur la modélisation neuronale (CNeuroMod). Je suis en outre chercheur-boursier sénior du Fonds de recherche du Québec - Santé (FRQS), membre de l'Alliance québécoise pour l'unification des neurosciences et de l'IA (UNIQUE) et directeur scientifique de l'Unité de neuro-imagerie fonctionnelle (UNF) du CRIUGM.