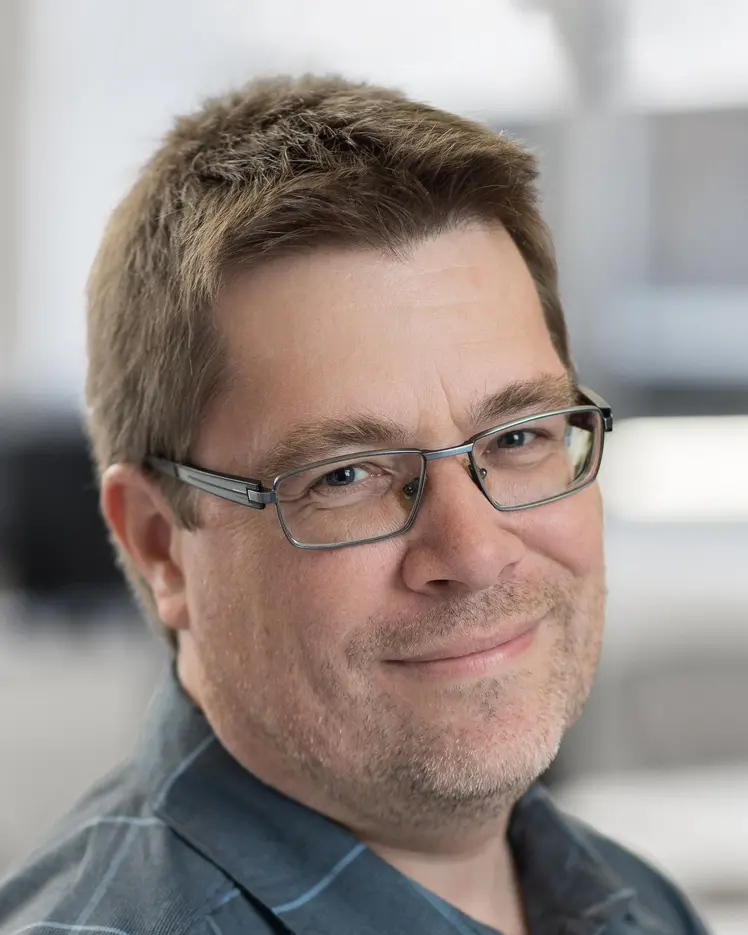
Pascal Vincent
Biographie
Pascal Vincent est chercheur à Meta (FAIR, Fundamental IA Research), professeur associé au Département d'informatique et de recherche opérationnelle (DIRO) de l'Université de Montréal, membre fondateur de Mila – Institut québécois d’intelligence artificielle et chercheur associé à l'Institut canadien de recherches avancées (CIFAR, programme Apprentissage automatique, apprentissage biologique).
Ses recherches sur les principes et les algorithmes de l'apprentissage par représentation l'ont amené à développer plusieurs idées fondamentales qui sont devenues des éléments clés du succès des méthodes d'apprentissage profond. Parmi ses travaux les plus influents, il est coauteur de l'article fondateur sur les modèles de langage neuronaux « A Neural Probabilistic Language Model » (Bengio et al., 2013), qui a jeté les bases de tous les modèles de langage fondés sur les réseaux de neurones artificiels. Son travail sur les auto-encodeurs de débruitage (Vincent et al., 2008, 2010) a été le premier à proposer la tâche prétexte de remplir des blancs artificiellement introduits dans le but d'apprendre des représentations utiles dans n'importe quelle modalité, un précurseur de ce que l'on appelle aujourd'hui « l'apprentissage autosupervisé ». En 2011, il a développé le principe du denoising score matching (P. Vincent, « A connection between score matching and denoising autoencoders », Neural Computation, 2011), qui est maintenant couramment utilisé pour former des modèles génératifs basés sur la diffusion. Ses recherches actuelles se concentrent sur de nouvelles théories et de nouveaux algorithmes pour l'apprentissage de la représentation afin de permettre une généralisation robuste en dehors de la distribution.