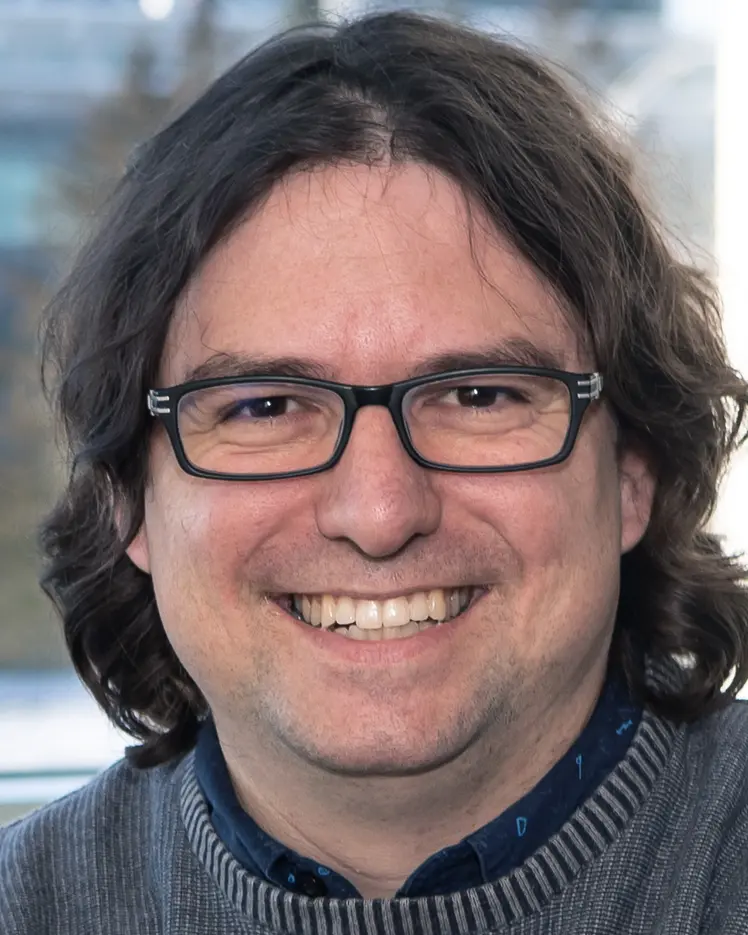
Pascal Germain
Biographie
Pascal Germain est professeur adjoint au Département d’informatique et de génie logiciel de l’Université Laval et chercheur en apprentissage automatique. Il a obtenu un doctorat en informatique de l'Université Laval en 2015, sous la direction de François Laviolette et de Mario Marchand. Il a ensuite poursuivi ses travaux de recherche en France pendant quatre ans au sein de l’Institut national de recherche en sciences et technologies du numérique (Inria), d'abord comme postdoctorant dans l'équipe du projet SIERRA de Francis Bach, puis comme chargé de recherche et membre de l'équipe du projet MODAL. Il a aussi été membre affilié et enseignant au Département de mathématiques de l'Université de Lille. De retour à son alma mater en tant que professeur adjoint depuis 2019, il y est membre du Centre de recherche en données massives (CRDM) et de l'Institut intelligence et données (IID). Il est également membre académique associé de Mila – Institut québécois d’intelligence artificielle. Ses domaines de recherche comprennent la théorie statistique de l’apprentissage automatique, l'apprentissage par transfert et l'apprentissage de prédicteurs interprétables.