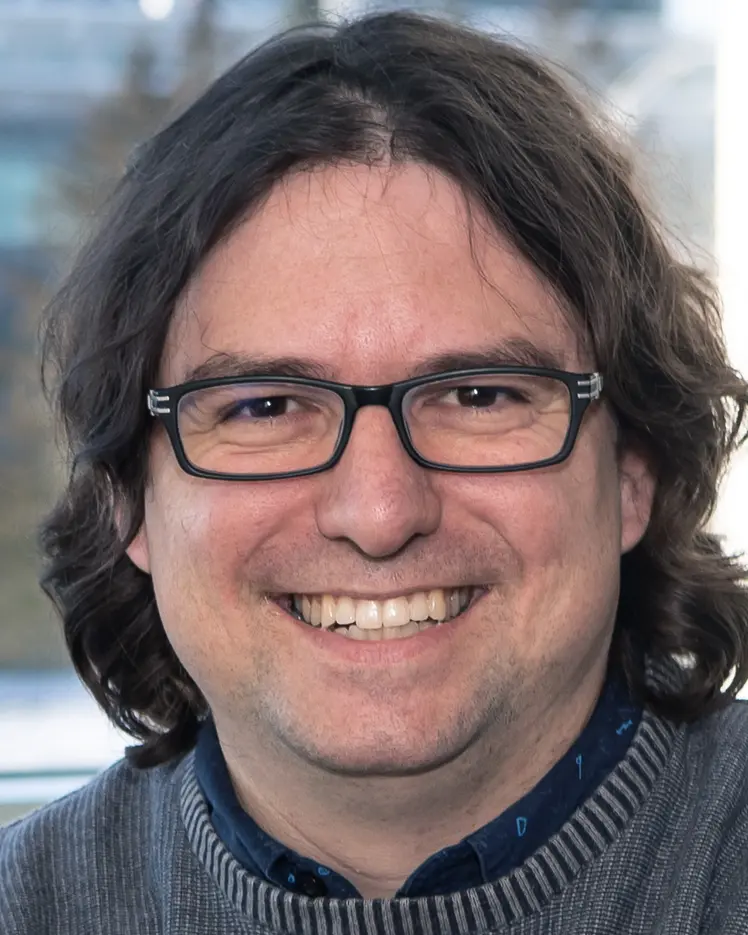
Pascal Germain
Biography
Pascal Germain is an assistant professor in the Computer Science and Software Engineering Department at Université Laval, and a Canada CIFAR AI Chair. He received his PhD in computer science from Université Laval in 2015 under the supervision of François Laviolette and Mario Marchand. He then pursued his research at the INRIA in France for four years, first as a postdoctoral fellow with Francis Bach’s SIERRA project team, then as a research fellow in the MODAL project team. Germain was also an affiliate member and lecturer in the Mathematics Department at the University of Lille. He returned to his alma mater, Université Laval, in 2019 to take on the role of assistant professor.
He is currently a member of Université Laval’s Big Data Research Centre (CRDM) and Institute Intelligence and Data (IID), as well as an associate academic member of Mila – Quebec Artificial Intelligence Institute. His research interests include machine learning, in particular statistical machine learning theory, transfer learning and interpretable predictor learning.